
如果你也在 怎样代写金融数学Financial Mathematics MT4551这个学科遇到相关的难题,请随时右上角联系我们的24/7代写客服。金融数学Financial Mathematics法国数学家Louis Bachelier被认为是第一部关于数学金融的学术著作的作者,发表于1900年。但数学金融作为一门学科出现在20世纪70年代,是在费舍尔-布莱克、迈伦-斯科尔斯和罗伯特-默顿关于期权定价理论的工作之后。数学投资起源于数学家爱德华-索普的研究,他利用统计方法首先发明了21点中的算牌,然后将其原理应用于现代系统投资。
金融数学Financial Mathematics该学科与金融经济学学科有着密切的关系,金融经济学涉及到金融数学中的许多基础理论。一般来说,数学金融学会以观察到的市场价格为输入,推导和扩展数学或数字模型,而不一定与金融理论建立联系。需要的是数学上的一致性,而不是与经济理论的兼容性。因此,例如,金融经济学家可能会研究一家公司可能有某种股价的结构性原因,而金融数学家可能会把股价作为一个给定值,并试图使用随机微积分来获得股票的相应衍生品价值。见。期权的估价;金融建模;资产定价。无套利定价的基本定理是数学金融学的关键定理之一,而布莱克-斯科尔斯方程和公式是其中的关键结果。
金融数学Financial Mathematics代写,免费提交作业要求, 满意后付款,成绩80\%以下全额退款,安全省心无顾虑。专业硕 博写手团队,所有订单可靠准时,保证 100% 原创。 最高质量的金融数学Financial Mathematics作业代写,服务覆盖北美、欧洲、澳洲等 国家。 在代写价格方面,考虑到同学们的经济条件,在保障代写质量的前提下,我们为客户提供最合理的价格。 由于作业种类很多,同时其中的大部分作业在字数上都没有具体要求,因此金融数学Financial Mathematics作业代写的价格不固定。通常在专家查看完作业要求之后会给出报价。作业难度和截止日期对价格也有很大的影响。
avatest™帮您通过考试
avatest™的各个学科专家已帮了学生顺利通过达上千场考试。我们保证您快速准时完成各时长和类型的考试,包括in class、take home、online、proctor。写手整理各样的资源来或按照您学校的资料教您,创造模拟试题,提供所有的问题例子,以保证您在真实考试中取得的通过率是85%以上。如果您有即将到来的每周、季考、期中或期末考试,我们都能帮助您!
在不断发展的过程中,avatest™如今已经成长为论文代写,留学生作业代写服务行业的翘楚和国际领先的教育集团。全体成员以诚信为圆心,以专业为半径,以贴心的服务时刻陪伴着您, 用专业的力量帮助国外学子取得学业上的成功。
•最快12小时交付
•200+ 英语母语导师
•70分以下全额退款
想知道您作业确定的价格吗? 免费下单以相关学科的专家能了解具体的要求之后在1-3个小时就提出价格。专家的 报价比上列的价格能便宜好几倍。
我们在数学Mathematics代写方面已经树立了自己的口碑, 保证靠谱, 高质且原创的数学Mathematics代写服务。我们的专家在金融数学Financial Mathematics代写方面经验极为丰富,各种运金融数学Financial Mathematics相关的作业也就用不着 说。

数学代写|金融数学代写Financial Mathematics代考|Extraneous Signals: Trading Volume, Volatility, etc.
The trading algorithms that are presented in this chapter thus far focus on the price and return behavior of an equity. In pairs trading, we considered the behavior of price-return of a related equity to decide when to buy one and sell the other. But there is also information, as noted in Section 4.3, present in the trading volume. The model discussed there relates the volume to volatility. If there is no variation in the information flow about an equity, there should not be any correlation between volume traded and the return volatility. If the correlation exists, we could indirectly infer that there is information and we may want to exploit that for trading. There is a vast literature in finance studying the relationship between trading volume and serial correlation in returns. We briefly review some select studies and provide an illustrative example with some possible algorithms.
Blume, Easley and O’Hara (1994) [45] investigate the informational role of volume. If the impounding of information into the stock price is not immediate, volume may provide information about the evolving process of a security’s return. The model discussed in Section $4.3$ by Tauchen and Pitts (1983) [311] and the associated empirical tests clearly document the strong relationship between volume and the absolute return. “But why such a pattern exists or even how volume evolves in markets is not clear.” Recall that the model assumes that the information content affects both the return and the volume specifications; yet the correlation between the return and volume can be shown to be zero. If the information content can be studied through the sequence of security prices and the associated volume, it may provide insights into the inefficiency in the market and how statistical arbitrage rules can exploit the inefficiency especially if there is an empirical lead-lag relationship. The quality of traders information can be captured best by combining price change with volume change. It is shown that the relationship between volume and price is in the form of V-shape thus indicating a non-linear relationship. Both ‘bad’ and ‘good’ information about the stock is likely to result in higher volume of trade.
Campbell, Grossman and Wang (1993) [65] also explore the relationship between volume and returns; volume information is used to distinguish between price movements that occur due to publicly available information and those that reflect changes in expected returns. It is predicted that price changes with high volume tend to be reversed and this relationship may not hold on days with low volume. Llorente, Michaely, Saar and Wang (2002) [246] extend this work by postulating that the market generally consists of liquidity and speculative traders. In periods of high volume but speculative trading, return autocorrelation tends to be positive and if it is liquidity trading, return autocorrelation tends to be negative. In the former case, returns are less likely to exhibit a reversal. Defining the volume turnover $(V)$ as the ratio of number of shares traded to the total number of outstanding shares, the following regression model is fit for each stock:
$$
r_{t}=\beta_{0}+\beta_{1} r_{t-1}+\beta_{2} V_{t-1} \cdot r_{t-1}+a_{t} .
$$
数学代写|金融数学代写Financial Mathematics代考|An Illustrative Example
The methodology discussed in the last section on price-based and volume-based filters on low frequency data can be extended to high frequency data as well. The key issue with the volume data is to come up with proper standardization to identify abnormal level of activity in a high frequency setting. The intra-day seasonality and the overall increase or decrease in inter-day volumes need to be tracked carefully to study and fully exploit the deviations. We highlight the practical issues involved using 30-min price-based data for Treasury yields from June 8, 2006 to August 29, $2013 .$ This can be taken as medium-frequency data. The price bars consist of high, low, open and close prices and the volume traded during the interval are considered. Because of the aggregated nature of the data, the traditional time series methods can be readily used to study the trend in price and volume series. Daily data is just a snapshot at 4 p.m. and the total volume for the day is the sum of all 30 -min volumes since the previous day.
Let $p_{t . m}$ be the log price in the $m$ th interval of the day ‘ $t$ ‘. Note that ‘ $m$ ‘ indexes the 30 -min interval in a day; the value of $m$ can range from 1 to 13. Let $r_{t . m}=p_{t . m}-p_{t . m-1}$ be the return and let $v_{t . m}$ be the log volume in that interval. We define volatility within the $m$ th time unit based on the price bar data as,
$$
\hat{\sigma}{t . m}^{2}=0.5\left[\ln \left(H{t . m}\right)-\ln \left(L_{t . m}\right)\right]^{2}-0.386\left[\ln \left(C_{t . m}\right)-\ln \left(\mathrm{O}_{t . m}\right)\right]^{2}
$$
similar to a measure defined for the daily data in Section 4.5.

金融数学代写
数学代写|金融数学代写Financial Mathematics代考|Extraneous Signals: Trading Volume, Volatility, etc.
到目前为止,本章介绍的交易算法主要关注股票的价格和回报行为。在配对交易中,我们考虑了相关股票的价 格回报行为来决定何时买入和卖出另一只。但是,如第 $4.3$ 节所述,交易量中也存在信息。那里讨论的模型将 交易量与波动性联系起来。如果有关股票的信息流没有变化,则交易量与回报波动之间不应存在任何相关性。 如果存在相关性,我们可以间接推断存在信息,我们可能希望利用该信息进行交易。有大量的金融文献研究交 易量和收益的序列相关性之间的关系。
Blume、Easley 和 O’Hara (1994) [45] 研究了音量的信息作用。如果不能立即将信息纳入股票价格,则交易 量可能会提供有关证券回报演变过程的信息。部分讨论的模型4.3Tauchen 和 Pitts (1983) [311] 以及相关的 实证检验清楚地证明了交易量和绝对回报之间的密切关系。“但为什么存在这种模式,甚至市场交易量如何演变 都不清楚。”回想一下,该模型假设信息内容同时影响回报和数量规格;然而,回报和交易量之间的相关性可以 显示为露。如果可以通过证券价格和相关交易量的顺序来研究信息内容,则可以深入了解市场的低效率,以及 统计套利规则如何利用低效率,尤其是在存在经验领先-滞后关系的情况下。通过将价格变化与交易量变化结合 起来,可以最好地捕捉交易者信息的质量。结果表明,成交量和价格之间的关系呈 $V$ 形,因此表明了一种非线性 关系。关于股票的“坏”和“好”信息都可能导致交易量增加。
Campbell、Grossman 和 Wang (1993) [65]也探讨了交易量和收益之间的关系;交易量信息用于区分由 于公开信息而发生的价格变动和反映预期收益变化的价格变动。据预测,高成交量的价格变化往往会逆转,这 种关系在成交量低的日子可能不成立。Llorente、Michaely、Saar 和 Wang (2002) [246] 通过假设市场通 常由流动性和投机交易者组成来扩展这项工作。在交易量大但投机交易的时期,收益自相关往往是正的,如果 是流动性交易,收益自相关往往是负的。在前一种情况下,回报不太可能出现逆转。定义成交量 $(V)$ 作为交易 股数与流通股总数的比率,以下回归模型适用于每只股票:
$$
r_{t}=\beta_{0}+\beta_{1} r_{t-1}+\beta_{2} V_{t-1} \cdot r_{t-1}+a_{t} .
$$
数学代写|金融数学代写Financial Mathematics代考|An Illustrative Example
上一节中讨论的基于价格和基于数量的低频数据过滤器的方法也可以扩展到高频数据。体积数据的关键问题是 提出适当的标准化来识别高频设置中的异常活动水平。需要仔细跟踪日内案节性和日间交易量的整体增减情
况,以研究和充分利用偏差。我们强调使用 2006 年 6 月 8 日至 8 月 29 日的 30 分钟基于价格的美国国债收 益率数据所涉及的实际问题,2013. 这可以作为中频数据。价格条由最高价、最低价、开盘价和收盘价组成,并 考虑了区间内的交易量。由于数据的聚合性质,传统的时间序列方法可以很容易地用于研究价格和数量序列的 趋势。每日数据只是下午 4 点的快照,当天的总交易量是自前一天以来所有 30 分钟交易量的总和。 让 $r_{t . m}=p_{t . m}-p_{t . m-1}$ 成为回报并让 $v_{t . m}$ 是该间隔内的日志量。我们将波动性定义为 $m$ 基于价格柱数据的时 间单位为,
$$
\hat{\sigma} t . m^{2}=0.5\left[\ln (H t . m)-\ln \left(L_{t . m}\right)\right]^{2}-0.386\left[\ln \left(C_{t . m}\right)-\ln \left(\mathrm{O}_{t . m}\right)\right]^{2}
$$
类似于第 $4.5$ 节中为每日数据定义的度量。
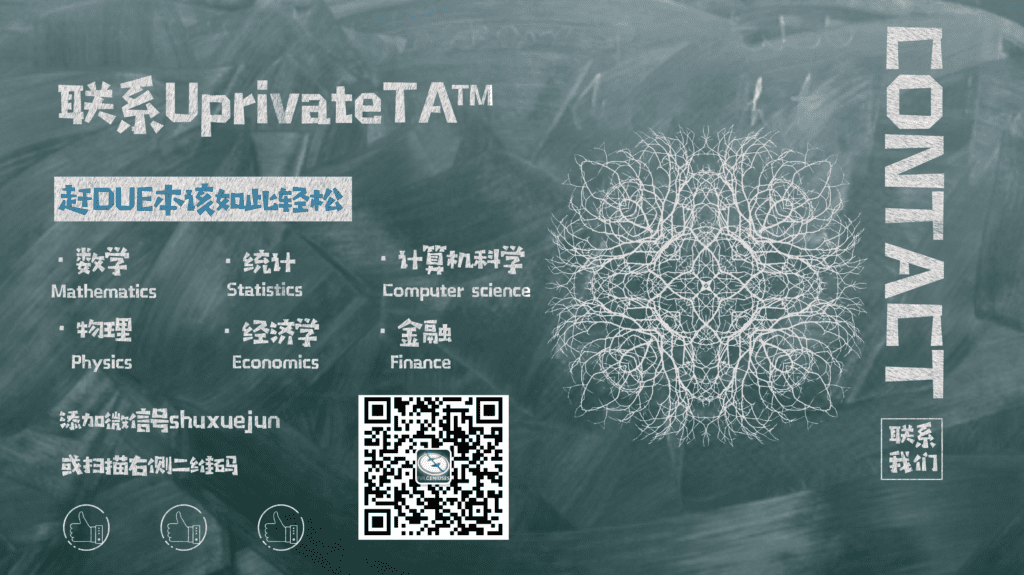
数学代写|金融数学代写Financial Mathematics代考 请认准UprivateTA™. UprivateTA™为您的留学生涯保驾护航。
微观经济学代写
微观经济学是主流经济学的一个分支,研究个人和企业在做出有关稀缺资源分配的决策时的行为以及这些个人和企业之间的相互作用。my-assignmentexpert™ 为您的留学生涯保驾护航 在数学Mathematics作业代写方面已经树立了自己的口碑, 保证靠谱, 高质且原创的数学Mathematics代写服务。我们的专家在图论代写Graph Theory代写方面经验极为丰富,各种图论代写Graph Theory相关的作业也就用不着 说。
线性代数代写
线性代数是数学的一个分支,涉及线性方程,如:线性图,如:以及它们在向量空间和通过矩阵的表示。线性代数是几乎所有数学领域的核心。
博弈论代写
现代博弈论始于约翰-冯-诺伊曼(John von Neumann)提出的两人零和博弈中的混合策略均衡的观点及其证明。冯-诺依曼的原始证明使用了关于连续映射到紧凑凸集的布劳威尔定点定理,这成为博弈论和数学经济学的标准方法。在他的论文之后,1944年,他与奥斯卡-莫根斯特恩(Oskar Morgenstern)共同撰写了《游戏和经济行为理论》一书,该书考虑了几个参与者的合作游戏。这本书的第二版提供了预期效用的公理理论,使数理统计学家和经济学家能够处理不确定性下的决策。
微积分代写
微积分,最初被称为无穷小微积分或 “无穷小的微积分”,是对连续变化的数学研究,就像几何学是对形状的研究,而代数是对算术运算的概括研究一样。
它有两个主要分支,微分和积分;微分涉及瞬时变化率和曲线的斜率,而积分涉及数量的累积,以及曲线下或曲线之间的面积。这两个分支通过微积分的基本定理相互联系,它们利用了无限序列和无限级数收敛到一个明确定义的极限的基本概念 。
计量经济学代写
什么是计量经济学?
计量经济学是统计学和数学模型的定量应用,使用数据来发展理论或测试经济学中的现有假设,并根据历史数据预测未来趋势。它对现实世界的数据进行统计试验,然后将结果与被测试的理论进行比较和对比。
根据你是对测试现有理论感兴趣,还是对利用现有数据在这些观察的基础上提出新的假设感兴趣,计量经济学可以细分为两大类:理论和应用。那些经常从事这种实践的人通常被称为计量经济学家。
MATLAB代写
MATLAB 是一种用于技术计算的高性能语言。它将计算、可视化和编程集成在一个易于使用的环境中,其中问题和解决方案以熟悉的数学符号表示。典型用途包括:数学和计算算法开发建模、仿真和原型制作数据分析、探索和可视化科学和工程图形应用程序开发,包括图形用户界面构建MATLAB 是一个交互式系统,其基本数据元素是一个不需要维度的数组。这使您可以解决许多技术计算问题,尤其是那些具有矩阵和向量公式的问题,而只需用 C 或 Fortran 等标量非交互式语言编写程序所需的时间的一小部分。MATLAB 名称代表矩阵实验室。MATLAB 最初的编写目的是提供对由 LINPACK 和 EISPACK 项目开发的矩阵软件的轻松访问,这两个项目共同代表了矩阵计算软件的最新技术。MATLAB 经过多年的发展,得到了许多用户的投入。在大学环境中,它是数学、工程和科学入门和高级课程的标准教学工具。在工业领域,MATLAB 是高效研究、开发和分析的首选工具。MATLAB 具有一系列称为工具箱的特定于应用程序的解决方案。对于大多数 MATLAB 用户来说非常重要,工具箱允许您学习和应用专业技术。工具箱是 MATLAB 函数(M 文件)的综合集合,可扩展 MATLAB 环境以解决特定类别的问题。可用工具箱的领域包括信号处理、控制系统、神经网络、模糊逻辑、小波、仿真等。