
如果你也在 怎样代写假设检验Hypothesis Testing MA121这个学科遇到相关的难题,请随时右上角联系我们的24/7代写客服。假设检验Hypothesis Testing是一种统计推断的形式,它使用来自样本的数据来得出关于一个群体参数或一个群体概率分布的结论。首先,对该参数或分布做出一个暂定的假设。
假设检验Hypothesis Testing虽然在20世纪初得到普及,但早期的形式在1700年代就被使用了。第一次使用被认为是John Arbuthnot(1710年),随后是Pierre-Simon Laplace(1770年代),在分析人类出生时的性别比时使用;见§ 人类性别比。现代意义检验主要是卡尔-皮尔逊(P值,皮尔逊的卡方检验)、威廉-西利-戈塞特(学生的t分布)和罗纳德-费雪(”无效假设”,方差分析,”意义检验”)的产物,而假设检验是由耶日-奈曼和埃贡-皮尔逊(卡尔的儿子)开发的。罗纳德-费舍尔作为贝叶斯主义者开始了他的统计生涯(Zabell 1992),但费舍尔很快就对其中的主观性(即在确定先验概率时使用冷漠原则)感到失望,并试图为归纳推理提供一种更 “客观 “的方法。
假设检验Hypothesis Testing代写,免费提交作业要求, 满意后付款,成绩80\%以下全额退款,安全省心无顾虑。专业硕 博写手团队,所有订单可靠准时,保证 100% 原创。最高质量的假设检验Hypothesis Testing作业代写,服务覆盖北美、欧洲、澳洲等 国家。 在代写价格方面,考虑到同学们的经济条件,在保障代写质量的前提下,我们为客户提供最合理的价格。 由于作业种类很多,同时其中的大部分作业在字数上都没有具体要求,因此假设检验Hypothesis Testing作业代写的价格不固定。通常在专家查看完作业要求之后会给出报价。作业难度和截止日期对价格也有很大的影响。
avatest™帮您通过考试
avatest™的各个学科专家已帮了学生顺利通过达上千场考试。我们保证您快速准时完成各时长和类型的考试,包括in class、take home、online、proctor。写手整理各样的资源来或按照您学校的资料教您,创造模拟试题,提供所有的问题例子,以保证您在真实考试中取得的通过率是85%以上。如果您有即将到来的每周、季考、期中或期末考试,我们都能帮助您!
在不断发展的过程中,avatest™如今已经成长为论文代写,留学生作业代写服务行业的翘楚和国际领先的教育集团。全体成员以诚信为圆心,以专业为半径,以贴心的服务时刻陪伴着您, 用专业的力量帮助国外学子取得学业上的成功。
•最快12小时交付
•200+ 英语母语导师
•70分以下全额退款
想知道您作业确定的价格吗? 免费下单以相关学科的专家能了解具体的要求之后在1-3个小时就提出价格。专家的 报价比上列的价格能便宜好几倍。
我们在统计Statistics代写方面已经树立了自己的口碑, 保证靠谱, 高质且原创的统计Statistics代写服务。我们的专家在假设检验Hypothesis Testing代写方面经验极为丰富,各种假设检验Hypothesis Testing相关的作业也就用不着说。

数据科学代写|假设检验代考Hypothesis Testing代考|Problems with Assuming Normality
To begin, distributions are never normal. For some this seems obvious, hardly worth mentioning. But an aphorism given by Cramér (1946) and attributed to the mathematician Poincaré remains relevant: “Everyone believes in the [normal] law of errors, the experimenters because they think it is a mathematical theorem, the mathematicians because they think it is an experimental fact.” Granted, the normal distribution is the most important distribution in all of statistics. But in terms of approximating the distribution of any continuous distribution, it can fail to the point that practical problems arise, as will become evident at numerous points in this book. To believe in the normal distribution implies that only two numbers are required to tell us everything about the probabilities associated with a random variable: the population mean $\mu$ and population variance $\sigma^2$. Moreover, assuming normality implies that distributions must be symmetric.
Of course, nonnormality is not, by itself, a disaster. Perhaps a normal distribution provides a good approximation of most distributions that arise in practice, and of course there is the central limit theorem, which tells us that under random sampling, as the sample size gets large, the limiting distribution of the sample mean is normal. Unfortunately, even when a normal distribution provides a good approximation to the actual distribution being studied (as measured by the Kolmogorov distance function, described later), practical problems arise. Also, empirical investigations indicate that departures from normality that have practical importance are rather common in applied work (e.g., M. Hill and Dixon, 1982; Micceri, 1989; Wilcox, 1990a). Even over a century ago, Karl Pearson and other researchers were concerned about the assumption that observations follow a normal distribution (e.g., Hand, 1998, p. 649). In particular, distributions can be highly skewed, they can have heavy tails (tails that are thicker than a normal distribution), and random samples often have outliers (unusually large or small values among a sample of observations). Outliers and heavy-tailed distributions are a serious practical problem because they inflate the standard error of the sample mean, so power can be relatively low when comparing groups. Modern robust methods provide an effective way of dealing with this problem. Fisher (1922), for example, was aware that the sample mean could be inefficient under slight departures from normality.
A classic way of illustrating the effects of slight departures from normality is with the contaminated, or mixed, normal distribution (Tukey, 1960). Let $X$ be a standard normal random variable having distribution $\Phi(x)=P(X \leq x)$. Then for any constant $K>0, \Phi(x / K)$ is a normal distribution with standard deviation $K$. Let $\epsilon$ be any constant, $0 \leq \epsilon \leq 1$. The contaminated normal distribution is
$$
H(x)=(1-\epsilon) \Phi(x)+\epsilon \Phi(x / K),
$$
which has mean 0 and variance $1-\epsilon+\epsilon K^2$. (Stigler, 1973, finds that the use of the contaminated normal dates back at least to Newcomb, 1896.) In other words, the contaminated normal arises by sampling from a standard normal distribution with probability $1-\epsilon$; otherwise sampling is from a normal distribution with mean 0 and standard deviation $K$.
数据科学代写|假设检验代考Hypothesis Testing代考|Transformations
Transforming data has practical value in a variety of situations. Emerson and Stoto (1983) provide a fairly elementary discussion of the various reasons one might transform data and how it can be done. The only important point here is that simple transformations can fail to deal effectively with outliers and heavy-tailed distributions. For example, the popular strategy of taking logarithms of all the observations does not necessarily reduce problems due to outliers, and the same is true when using Box-Cox transformations instead (e.g., Rasmussen, 1989; Doksum and Wong, 1983). Other concerns were expressed by G. L. Thompson and Amman (1990). Better strategies are described in subsequent chapters.
Perhaps it should be noted that when using simple transformations on skewed data, if inferences are based on the mean of the transformed data, then attempts at making inferences about the mean of the original data, $\mu$, have been abandoned. That is, if the mean of the transformed data is computed and we transform back to the original data, in general we do not get an estimate of $\mu$.

假设检验代写
数据科学代写|假设检验代考Hypothesis Testing代考|Problems with Assuming
首先,分布从来都不是正常的。对于某些人来说,这似乎很明显,几平不值得一提。但是,Cramér (1946) 给出的并归因于数学 家 Poincaré 的格言仍然相关: “每个人都相信[正常] 误差定律,实验者因为他们认为这是一个数学定理,数学家因为他们认为这 息:总体均值 $\mu$ 和总体方差 $\sigma^2$. 此外,假设正态性意味着分布必须是对称的。
当然,非正态性本身并不是一场灾难。也许正态分布可以很好地逼近实践中出现的大多数分布,当然还有中心极限定理,它告诉我 们在随机柚样下,随差样本量变大,样本均值的极限分布是正态的. 不幸的是,即使正态分布提供了对正在研究的实际分布的良好 近似(如通过Kolmogorov 距离函数测量,稍后描述),也会出现实际问题。此外,实证调龺表明,具有实际重要性的偏离常态 在应用工作中相当普遍(例如,M. Hill 和 Dixon,1982;Micceri,1989;Wilcox,1990a)。即使在一个多世纪以前,Karl Pearson 和其他研究人员担心观究结果服从正态分布的假设(例如,Hand, 1998, p. 649)。特别是,分布可能高度偏斜,它们 可能有重尾(比正态分布更粗的尾),并且随机样本通常有异常值(观察样本中的值异常大或小)。异常值和重尾分布是一个严重 的实际问题,因为它们夸大了样本均值的标准误差,因此在比较组时功效可能相对较低。现代稳健的方法是供了一种有效的方法来 解决这个问题。例如,Fisher (1922) 意识到样本均值在轻微偏离正态性的情况下可能效率低下。分布可能是高度偏斜的,它们可 能有重尾(比正态分布更粗的尾),并且随机样本通常有异常值(观察样本中的值异常大或小)。异常值和重尾分布是一个严重的 实际问题,因为它们夸大了样本均值的标准误差,因此在比较组时功效可能相对较低。现代稳健的方法是供了一种有效的方法来解 决这个问题。例如,Fisher (1922) 竟吅到样本均值在轻微偏离正圭性的情况下可能效率低下。分布可能是高度偏䣄的,它们可能 际问题,因为它们夸大了样本均值的标准吴差,因此在比较组时功效可能相对较低,现代稳健的方法㪚供了一种有效的方法来解决 这个问题。例如,Fisher (1922) 意识到样本均值在轻微偏离正态性的情况下可能效率低下。异常值和重尾分布是一个严重的实际 问题,因为它们玄大了样本均值的标准误差,因此在比较组时功效可能相对较低。现代稳健的方法提供了一种有效的方法来解决这 个问题。例如,Fisher (1922) 意吅到样本均值在轻微偏离正态性的情况下可能效率低下。异常值和重尾分布是个严重的实际问 题,因为它们夸大了样本均值的标准误差,因此在比较组时功效可能相对较低。现代稳健的方法提供了一种有效的方法来解决这个 问题。例如,Fisher (1922) 意识到样本均值在轻微偏离正态性的情况下可能效率低下。
说明轻微偏离正态的影响的经典方法是使用受污染的或混合的正态分布(Tukey,1960)。让 $X$ 是具有分布的标准正态随机変量 $\Phi(x)=P(X \leq x)$. 那么对于任何常数 $K>0, \Phi(x / K)$ 是具有标准偏差的正态分布 $K .$ 让 $\epsilon$ 是任何常数, $0 \leq \epsilon \leq 1$. 污染正态
$$
H(x)=(1-\epsilon) \Phi(x)+\epsilon \Phi(x / K)
$$
均值为 0,方差为 $1-\epsilon+\epsilon K^2$. (Stigler,1973 年发现污染正态的使用至少可以追溯到 Newcomb,1896 年。) 换句话说, 污染正态是通过从具有概率的标准正态分布中抽样产生的 $1-\epsilon$; 否则采样来自均值为 0 和标准差的正态分布 $K$.
数据科学代写假设检验代考Hypothesis Testing代考|Transformations
转换数据在各种情况下都具有实用价值。Emerson 和 Stoto (1983) 对可能转换数据的各种原因以及如何完成数据进行了相当基 本的讨论。这里唯一重要的一点是,简单的转换可能无法有效地处理异常值和重尾分布。例如,对所有观察值取对数的流行策略并 不一定会減少由于异常值引起的问题,而在使用 Box-Cox 孪换时也是如此 (例如,Rasmussen, 1989;Doksum 和 Wong, 1983)。GL Thompson 和 Amman (1990) 表达了其他担忧。更好的策略将在后续章节中描述。
或许应该注意的是,在对倾斜数据使用简单䇂换时,如果推断是基于新换后数据的均值,则尝试对原始数据的均值进行推断, $\mu$ , 已被抛弃。也就是说,如果计算了转换数据的平均值,然后我们转换回原始数据,通常我们不会得到 $\mu$.
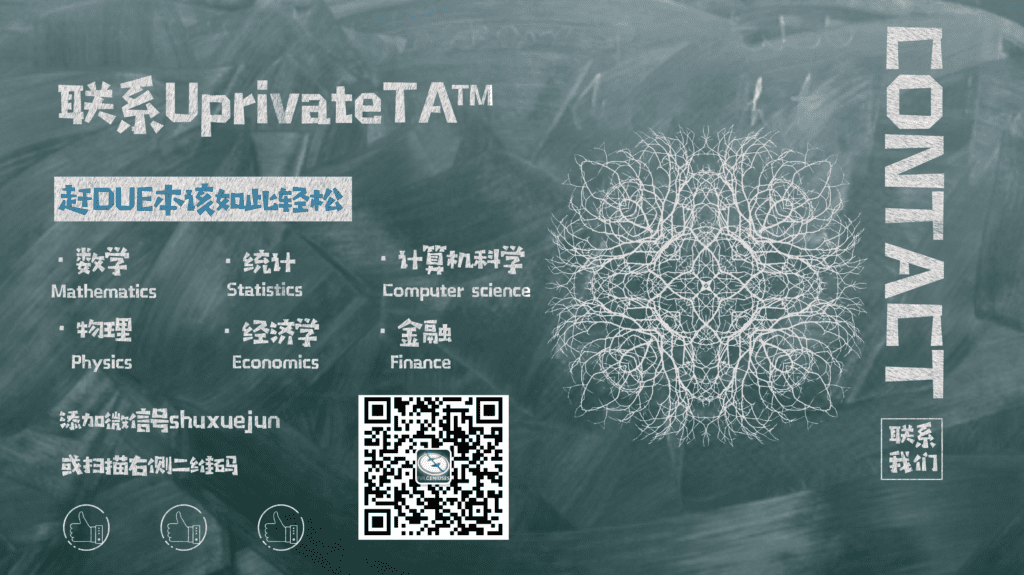
数据科学代写|假设检验代考Hypothesis Testing代考 请认准UprivateTA™. UprivateTA™为您的留学生涯保驾护航。
微观经济学代写
微观经济学是主流经济学的一个分支,研究个人和企业在做出有关稀缺资源分配的决策时的行为以及这些个人和企业之间的相互作用。my-assignmentexpert™ 为您的留学生涯保驾护航 在数学Mathematics作业代写方面已经树立了自己的口碑, 保证靠谱, 高质且原创的数学Mathematics代写服务。我们的专家在图论代写Graph Theory代写方面经验极为丰富,各种图论代写Graph Theory相关的作业也就用不着 说。
线性代数代写
线性代数是数学的一个分支,涉及线性方程,如:线性图,如:以及它们在向量空间和通过矩阵的表示。线性代数是几乎所有数学领域的核心。
博弈论代写
现代博弈论始于约翰-冯-诺伊曼(John von Neumann)提出的两人零和博弈中的混合策略均衡的观点及其证明。冯-诺依曼的原始证明使用了关于连续映射到紧凑凸集的布劳威尔定点定理,这成为博弈论和数学经济学的标准方法。在他的论文之后,1944年,他与奥斯卡-莫根斯特恩(Oskar Morgenstern)共同撰写了《游戏和经济行为理论》一书,该书考虑了几个参与者的合作游戏。这本书的第二版提供了预期效用的公理理论,使数理统计学家和经济学家能够处理不确定性下的决策。
微积分代写
微积分,最初被称为无穷小微积分或 “无穷小的微积分”,是对连续变化的数学研究,就像几何学是对形状的研究,而代数是对算术运算的概括研究一样。
它有两个主要分支,微分和积分;微分涉及瞬时变化率和曲线的斜率,而积分涉及数量的累积,以及曲线下或曲线之间的面积。这两个分支通过微积分的基本定理相互联系,它们利用了无限序列和无限级数收敛到一个明确定义的极限的基本概念 。
计量经济学代写
什么是计量经济学?
计量经济学是统计学和数学模型的定量应用,使用数据来发展理论或测试经济学中的现有假设,并根据历史数据预测未来趋势。它对现实世界的数据进行统计试验,然后将结果与被测试的理论进行比较和对比。
根据你是对测试现有理论感兴趣,还是对利用现有数据在这些观察的基础上提出新的假设感兴趣,计量经济学可以细分为两大类:理论和应用。那些经常从事这种实践的人通常被称为计量经济学家。
MATLAB代写
MATLAB 是一种用于技术计算的高性能语言。它将计算、可视化和编程集成在一个易于使用的环境中,其中问题和解决方案以熟悉的数学符号表示。典型用途包括:数学和计算算法开发建模、仿真和原型制作数据分析、探索和可视化科学和工程图形应用程序开发,包括图形用户界面构建MATLAB 是一个交互式系统,其基本数据元素是一个不需要维度的数组。这使您可以解决许多技术计算问题,尤其是那些具有矩阵和向量公式的问题,而只需用 C 或 Fortran 等标量非交互式语言编写程序所需的时间的一小部分。MATLAB 名称代表矩阵实验室。MATLAB 最初的编写目的是提供对由 LINPACK 和 EISPACK 项目开发的矩阵软件的轻松访问,这两个项目共同代表了矩阵计算软件的最新技术。MATLAB 经过多年的发展,得到了许多用户的投入。在大学环境中,它是数学、工程和科学入门和高级课程的标准教学工具。在工业领域,MATLAB 是高效研究、开发和分析的首选工具。MATLAB 具有一系列称为工具箱的特定于应用程序的解决方案。对于大多数 MATLAB 用户来说非常重要,工具箱允许您学习和应用专业技术。工具箱是 MATLAB 函数(M 文件)的综合集合,可扩展 MATLAB 环境以解决特定类别的问题。可用工具箱的领域包括信号处理、控制系统、神经网络、模糊逻辑、小波、仿真等。