
如果你也在 怎样代写凸分析和最优控制Convex Analysis and Optimal Control MATH4071这个学科遇到相关的难题,请随时右上角联系我们的24/7代写客服。凸分析和最优控制Convex Analysis and Optimal Control是变迁微积分的延伸,是一种用于推导控制政策的数学优化方法。该方法主要归功于Lev Pontryagin和Richard Bellman在20世纪50年代的工作,此前Edward J. McShane对变迁微积分做出了贡献。
凸分析和最优控制Convex Analysis and Optimal Control是数学优化的一个分支,它涉及在一段时间内为一个动态系统寻找一个控制,使目标函数得到优化。例如,动态系统可能是一个航天器,其控制装置与火箭推进器相对应,其目标可能是以最小的燃料消耗到达月球。或者动态系统可能是一个国家的经济,其目标是最小化失业;这种情况下的控制可能是财政和货币政策。
凸分析和最优控制Convex Analysis and Optimal Control代写,免费提交作业要求, 满意后付款,成绩80\%以下全额退款,安全省心无顾虑。专业硕 博写手团队,所有订单可靠准时,保证 100% 原创。最高质量的凸分析和最优控制Convex Analysis and Optimal Control作业代写,服务覆盖北美、欧洲、澳洲等 国家。 在代写价格方面,考虑到同学们的经济条件,在保障代写质量的前提下,我们为客户提供最合理的价格。 由于统计Statistics作业种类很多,同时其中的大部分作业在字数上都没有具体要求,因此凸分析和最优控制Convex Analysis and Optimal Control作业代写的价格不固定。通常在专家查看完作业要求之后会给出报价。作业难度和截止日期对价格也有很大的影响。
avatest™帮您通过考试
avatest™的各个学科专家已帮了学生顺利通过达上千场考试。我们保证您快速准时完成各时长和类型的考试,包括in class、take home、online、proctor。写手整理各样的资源来或按照您学校的资料教您,创造模拟试题,提供所有的问题例子,以保证您在真实考试中取得的通过率是85%以上。如果您有即将到来的每周、季考、期中或期末考试,我们都能帮助您!
在不断发展的过程中,avatest™如今已经成长为论文代写,留学生作业代写服务行业的翘楚和国际领先的教育集团。全体成员以诚信为圆心,以专业为半径,以贴心的服务时刻陪伴着您, 用专业的力量帮助国外学子取得学业上的成功。
•最快12小时交付
•200+ 英语母语导师
•70分以下全额退款
想知道您作业确定的价格吗? 免费下单以相关学科的专家能了解具体的要求之后在1-3个小时就提出价格。专家的 报价比上列的价格能便宜好几倍。

数学代写|凸分析和最优控制代写Convex Analysis and Optimal Control代考|New fundamentals of Young measure convergence
This paper presents a new, penetrating approach to Young measure convergence in an abstract, measure theoretical setting. It was started in $[12,13,14]$ and given its definitive shape in $[18,22]$. This approach is based on $K$-convergence, a device by which narrow convergence on $\mathcal{P}\left(\mathbf{R}^d\right)$ can be systematically transferred to Young measure convergence. Here $\mathcal{P}\left(\mathbf{R}^d\right)$ stands for the set of all probability measures on $\mathbf{R}^d$ (in the sequel, a much more general topological space $S$ is used instead of $\mathbf{R}^d$ ). Recall that in this context Young measures are measurable functions from an underlying finite measure space $(\Omega, \mathcal{A}, \mu)$ into $\mathcal{P}\left(\mathbf{R}^d\right)$. Recall also from [12], [13] (see also [24]) that $K$-convergence takes the following form when applied to Young measures (see Definition 3.1): A sequence $\left(\delta_k\right)$ of Young measures $K$-converges to a Young measure $\delta_0$ [notation: $\delta_k \stackrel{K}{\longrightarrow} \delta_0$ ] if for every subsequence $\left(\delta_{k_j}\right)$ of $\left(\delta_k\right)$ the following pointwise Cesaro-type convergence takes places
$$
\frac{1}{N} \sum_{j=1}^N \delta_{k_j}(\omega) \Rightarrow \delta_0(\omega) \text { as } N \rightarrow \infty
$$
at $\mu$-almost every point $\omega$ in $\Omega$. Here ” $\Rightarrow$ ” means classical narrow convergence on $\mathcal{P}\left(\mathbf{R}^d\right)$ (see Definition 2.1). As is shown much more completely in Proposition $3.6$ and Theorem $4.8$, the following fundamental relationship holds between Young measure convergence, denoted by ” $\Longrightarrow$ “, and $K$-convergence as just defined [18, Corollary $3.16]$ :
Theorem $1.1$ Let $\left(\delta_n\right)$ be a sequence of Young measures. The following are equivalent:
(a) $\delta_n \Longrightarrow \delta_0$
(b) Every subsequence $\left(\delta_{n^{\prime}}\right)$ of $\left(\delta_n\right)$ contains a further subsequence $\left(\delta_{n^{\prime \prime}}\right)$ such that $\delta_{n^{\prime \prime}} \stackrel{K}{\longrightarrow} \delta_0$
数学代写|凸分析和最优控制代写Convex Analysis and Optimal Control代考|Narrow convergence of probability measures
This section recapitulates some results on narrow convergence of probability measures on a metric space; cf. $[2,27,28,35,46]$. Let $S$ be a completely regular Suslin space, whose topology is denoted by $\tau$. On such a space there exists a metric $\rho$ whose topology $\tau_\rho$ is not stronger than $\tau$, with the property that the Borel $\sigma$-algebras $\mathcal{B}\left(S, \tau_\rho\right)$ and $\mathcal{B}(S, \tau)$ coincide. To see this, recall that in a completely regular space the points are separated by the collection $\mathcal{C}b(S, \tau)$ of all bounded continuous functions on $S$. Since $S$ is also Suslin, it follows by [32, III.32] that there exists a countable subset $\left(c_i\right)$ of $\mathcal{C}_b(S, \tau)$, with $\sup {x \in S}\left|c_i(x)\right|=1$ for each $i$, that still separates the points of $S$. A metric as desired is then given by $\rho(x, y):=\sum_{i=1}^{\infty} 2^{-i}\left|c_i(x)-c_i(y)\right|$. This is because $\tau_\rho \subset \tau$ is obvious and by another well-known property of Suslin spaces, the Borel $\sigma$-algebras $\mathcal{B}(S, \rho)$ and $\mathcal{B}(S, \tau)$ coincide [51, Corollary 2, p. 101]. Of course, if $S$ is a metrizable Suslin space to begin with, then for $\rho$ one can simply take any metric on $S$ that is compatible with $\tau$.
As a consequence of the above, we shall write from now on
$$
\mathcal{B}(S):=\mathcal{B}(S, \rho)=\mathcal{B}(S, \tau), \mathcal{P}(S):=\mathcal{P}(S, \rho)=\mathcal{P}(S, \tau)
$$
for respectively the Borel $\sigma$-algebra and the set of all probability measures on $(S, \mathcal{B}(S))$.

凸分析和最优控制代写
数学代写|凸分析和最优控制代写Convex Analysis and Optimal Control代考|New fundamentals of Young measure convergence
本文在抽象的测量理论设置中提出了一种新的、深入的方法来解决Young 测量收敛。它开始于 $[12,13,14]$ 并给出了它最终的形 状 $[18,22]$. 这种方法基于 $K$-convergence,一种窒收敛的装置 $\mathcal{P}\left(\mathbf{R}^d\right)$ 可以系统地转移到 Young 度量收敛。这里 $\mathcal{P}\left(\mathbf{R}^d\right)$ 代 表所有概率恻度的集合 $\mathbf{R}^d$ (在续集中,一个更一般的拓扑空间 $S$ 被用来代替 $\mathbf{R}^d$ )。回想一下,在这种情况下,Young 测度是来 自潜在有限测度空间的可测函数 $(\Omega, \mathcal{A}, \mu)$ 进入 $\mathcal{P}\left(\mathbf{R}^d\right)$. 还从 [12]、 [13] (另见 [24]) 中回想一下 $K$-convergence 在应用于 Young 度量时采用以下形式 (参见定义 3.1) : 序列 $\left(\delta_k\right)$ 杨氏措施 $K$-收敛到 Young 度量 $\delta_0\left[\right.$ 符昊: $\left.\delta_k \stackrel{K}{\longrightarrow} \delta_0\right]$ 如果对于每个子 序列 $\left(\delta_{k_j}\right)$ 的 $\left(\delta_k\right)$ 发生以下逐点 Cesaro 型收敛
$$
\frac{1}{N} \sum_{j=1}^N \delta_{k j}(\omega) \Rightarrow \delta_0(\omega) \text { as } N \rightarrow \infty
$$
Young 测度收敛之间存在以下基本关系,记为 ” $\Longrightarrow “$ , 和 $K$-刚刚定义的收敛[18,推论 $3.16]:$
定理1.1让 $\left(\delta_n\right)$ 是一系列 Young 度量。以下是等效的:
(a) $\delta_n \Longrightarrow \delta_0$
(b) 每个子序列 $\left(\delta_{n^{\prime}}\right)$ 的 $\left(\delta_n\right)$ 包含另一个子序列 $\left(\delta_{n^{\prime \prime}}\right)$ 这样 $\delta_{n^{\prime \prime}} \stackrel{K}{\longrightarrow} \delta_0$
数学代写|凸分析和最优控制代写Convex Analysis and Optimal Control代 考|Narrow convergence of probability measures
本节概括了一些关于概率度量在度量空间上的窂收敛的结果;参看。[2, 27, 28, 35, 46]. 让 $S$ 是 个个完全正则的 Suslin 空间,其 拓扑记为 $\tau$. 在这样的空间上存在一个度量 $\rho$ 谁的拓扑 $\tau_\rho$ 不强于 $\tau$ ,具有 Borel 的性质 $\sigma$-代数 $\mathcal{B}\left(S, \tau_\rho\right)$ 和 $\mathcal{B}(S, \tau)$ 重合。要看到这一 点,请回想一下,在完全规则的空间中,点被集合分开 $\mathcal{C} b(S, \tau)$ 所有有界连续函数 $S$. 自从 $S$ 也是 Suslin,它遈循 [32, III.32] 存在 一个可数子集 $\left(c_i\right)$ 的 $\mathcal{C}b(S, \tau)$ ,和sup $x \in S\left|c_i(x)\right|=1$ 对于每个 $i$ ,这仍然桀点分开 $S$. 然后根据需要给出一个度量 $\rho(x, y):=\sum{i=1}^{\infty} 2^{-i}\left|c_i(x)-c_i(y)\right|$. 这是因为 $\tau_\rho \subset \tau$ 是显而易见的,并且由 Suslin 空间的另一个著名属性 Borel $\sigma$-代数 $\mathcal{B}(S, \rho)$ 和 $\mathcal{B}(S, \tau)$ 重合 [51,推论 2, p。101]。当然,如果 $S$ 是 个可度量的 Suslin 空间,然后为 $\rho$ 可以简单地采用任何指标 $S$ 与 $\tau$.
综上所述,我们从现在开始写
$$
\mathcal{B}(S):=\mathcal{B}(S, \rho)=\mathcal{B}(S, \tau), \mathcal{P}(S):=\mathcal{P}(S, \rho)=\mathcal{P}(S, \tau)
$$
分别为 Borel $\sigma$-代数和所有概率度量的集合 $(S, \mathcal{B}(S))$.
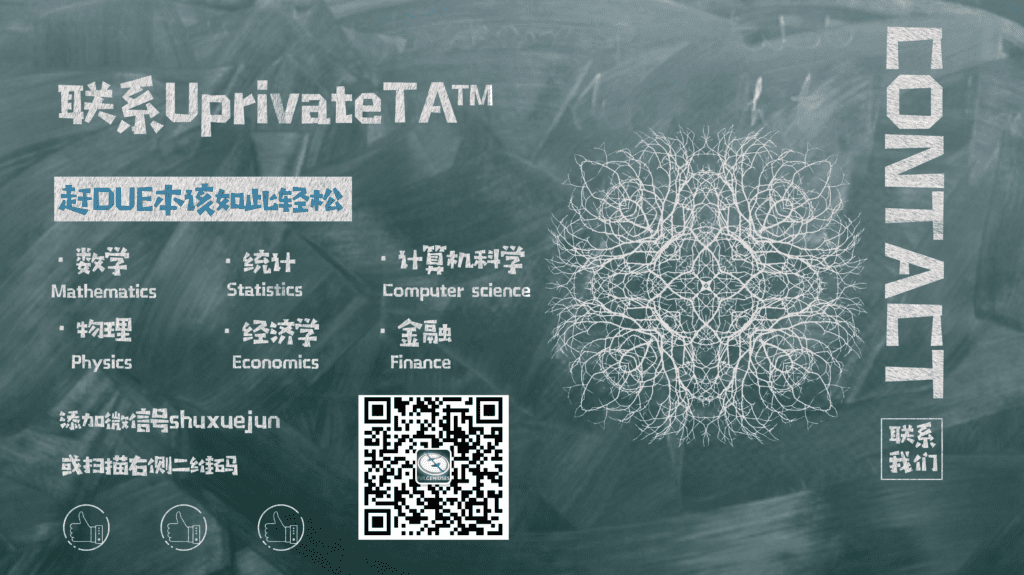
数学代写|凸分析和最优控制代写Convex Analysis and Optimal Control代考 请认准UprivateTA™. UprivateTA™为您的留学生涯保驾护航。
微观经济学代写
微观经济学是主流经济学的一个分支,研究个人和企业在做出有关稀缺资源分配的决策时的行为以及这些个人和企业之间的相互作用。my-assignmentexpert™ 为您的留学生涯保驾护航 在数学Mathematics作业代写方面已经树立了自己的口碑, 保证靠谱, 高质且原创的数学Mathematics代写服务。我们的专家在图论代写Graph Theory代写方面经验极为丰富,各种图论代写Graph Theory相关的作业也就用不着 说。
线性代数代写
线性代数是数学的一个分支,涉及线性方程,如:线性图,如:以及它们在向量空间和通过矩阵的表示。线性代数是几乎所有数学领域的核心。
博弈论代写
现代博弈论始于约翰-冯-诺伊曼(John von Neumann)提出的两人零和博弈中的混合策略均衡的观点及其证明。冯-诺依曼的原始证明使用了关于连续映射到紧凑凸集的布劳威尔定点定理,这成为博弈论和数学经济学的标准方法。在他的论文之后,1944年,他与奥斯卡-莫根斯特恩(Oskar Morgenstern)共同撰写了《游戏和经济行为理论》一书,该书考虑了几个参与者的合作游戏。这本书的第二版提供了预期效用的公理理论,使数理统计学家和经济学家能够处理不确定性下的决策。
微积分代写
微积分,最初被称为无穷小微积分或 “无穷小的微积分”,是对连续变化的数学研究,就像几何学是对形状的研究,而代数是对算术运算的概括研究一样。
它有两个主要分支,微分和积分;微分涉及瞬时变化率和曲线的斜率,而积分涉及数量的累积,以及曲线下或曲线之间的面积。这两个分支通过微积分的基本定理相互联系,它们利用了无限序列和无限级数收敛到一个明确定义的极限的基本概念 。
计量经济学代写
什么是计量经济学?
计量经济学是统计学和数学模型的定量应用,使用数据来发展理论或测试经济学中的现有假设,并根据历史数据预测未来趋势。它对现实世界的数据进行统计试验,然后将结果与被测试的理论进行比较和对比。
根据你是对测试现有理论感兴趣,还是对利用现有数据在这些观察的基础上提出新的假设感兴趣,计量经济学可以细分为两大类:理论和应用。那些经常从事这种实践的人通常被称为计量经济学家。
MATLAB代写
MATLAB 是一种用于技术计算的高性能语言。它将计算、可视化和编程集成在一个易于使用的环境中,其中问题和解决方案以熟悉的数学符号表示。典型用途包括:数学和计算算法开发建模、仿真和原型制作数据分析、探索和可视化科学和工程图形应用程序开发,包括图形用户界面构建MATLAB 是一个交互式系统,其基本数据元素是一个不需要维度的数组。这使您可以解决许多技术计算问题,尤其是那些具有矩阵和向量公式的问题,而只需用 C 或 Fortran 等标量非交互式语言编写程序所需的时间的一小部分。MATLAB 名称代表矩阵实验室。MATLAB 最初的编写目的是提供对由 LINPACK 和 EISPACK 项目开发的矩阵软件的轻松访问,这两个项目共同代表了矩阵计算软件的最新技术。MATLAB 经过多年的发展,得到了许多用户的投入。在大学环境中,它是数学、工程和科学入门和高级课程的标准教学工具。在工业领域,MATLAB 是高效研究、开发和分析的首选工具。MATLAB 具有一系列称为工具箱的特定于应用程序的解决方案。对于大多数 MATLAB 用户来说非常重要,工具箱允许您学习和应用专业技术。工具箱是 MATLAB 函数(M 文件)的综合集合,可扩展 MATLAB 环境以解决特定类别的问题。可用工具箱的领域包括信号处理、控制系统、神经网络、模糊逻辑、小波、仿真等。