
如果你也在 怎样代写随机微积分Stochastic Calculus MAST90059这个学科遇到相关的难题,请随时右上角联系我们的24/7代写客服。随机微积分Stochastic Calculus是数学的一个分支,对随机过程进行操作。它允许为随机过程的积分定义一个关于随机过程的一致的积分理论。这一领域是由日本数学家伊藤清在第二次世界大战期间创建并开始的。
随机微积分Stochastic Calculus MATH581 应用随机微积分的最著名的随机过程是维纳过程(为纪念诺伯特-维纳而命名),它被用来模拟路易-巴切莱特在1900年和阿尔伯特-爱因斯坦在1905年描述的布朗运动以及其他受随机力作用的粒子在空间的物理扩散过程。自20世纪70年代以来,维纳过程被广泛地应用于金融数学和经济学中,以模拟股票价格和债券利率的时间演变。
随机微积分Stochastic Calculus代写,免费提交作业要求, 满意后付款,成绩80\%以下全额退款,安全省心无顾虑。专业硕 博写手团队,所有订单可靠准时,保证 100% 原创。最高质量的随机微积分Stochastic Calculus作业代写,服务覆盖北美、欧洲、澳洲等 国家。 在代写价格方面,考虑到同学们的经济条件,在保障代写质量的前提下,我们为客户提供最合理的价格。 由于统计Statistics作业种类很多,同时其中的大部分作业在字数上都没有具体要求,因此随机微积分Stochastic Calculus作业代写的价格不固定。通常在经济学专家查看完作业要求之后会给出报价。作业难度和截止日期对价格也有很大的影响。
想知道您作业确定的价格吗? 免费下单以相关学科的专家能了解具体的要求之后在1-3个小时就提出价格。专家的 报价比上列的价格能便宜好几倍。
avatest™帮您通过考试
avatest™的各个学科专家已帮了学生顺利通过达上千场考试。我们保证您快速准时完成各时长和类型的考试,包括in class、take home、online、proctor。写手整理各样的资源来或按照您学校的资料教您,创造模拟试题,提供所有的问题例子,以保证您在真实考试中取得的通过率是85%以上。如果您有即将到来的每周、季考、期中或期末考试,我们都能帮助您!
在不断发展的过程中,avatest™如今已经成长为论文代写,留学生作业代写服务行业的翘楚和国际领先的教育集团。全体成员以诚信为圆心,以专业为半径,以贴心的服务时刻陪伴着您, 用专业的力量帮助国外学子取得学业上的成功。
•最快12小时交付
•200+ 英语母语导师
•70分以下全额退款

数学代写|随机微积分代写STOCHASTIC CALCULUS代考|Brownian motion
The stochastic process that we shall focus our attention on in this course is Brownian motion (BM) $W=\left(W_t\right){t \geq 0}$ (which is the building block for all stochastic calculus involving processes with continuous sample paths). Summarising it very briefly, BM is a continuous process with stationary, independent, normally distributed (or Gaussian) increments of mean zero and variance equal to the time elapsed during the increment. That is, for $0 \leq s \leq t<\infty$, the increment $W_t-W_s$ is distributed according to $W_t-W_s \sim \mathrm{N}(0, t-s)$ and is independent of $W_s$ (and, indeed, is independent of the entire history $\left(W_u\right){0 \leq u \leq s}$ ). Here, and elsewhere, we shall use the notation whereby $X \sim \mathrm{N}\left(m, s^2\right)$ denotes that a random variable $X$ is normally distributed with mean $m$ and variance $s^2$.
We shall give some rigorous definitions of BM shortly. BM has all the quintessential properties one could hope for: it is a martingale and a Markov process (as well as having continuous paths), and can also be thought of as the limit of a scaled and speeded-up random walk. However, the paths of BM are highly irregular, as we shall see; they are not differentiable, and are of infinite length over any finite time interval.
数学代写|随机微积分代写STOCHASTIC CALCULUS代考|Remarks on existence of BM
Remarks on existence of $\mathbf{B M}$. The existence of $\mathrm{BM}$ as a well-defined mathematical object is a non-trivial issue, but one that will not concern us – we shall assume the existence of BM, though we now make some brief remarks with references to where constructions of BM can be found.
One approach to showing the existence of $\mathrm{BM}$ is to write down what the finite-dimensional distributions of the process (based on stationarity, independence and normality of its increments) must be, and then construct a probability measure and a process on an appropriate measurable space in such a way that we obtain the prescribed finite-dimensional distributions.
This technique is a standard approach to constructing a Markov process (of which BM is an example), and can be lengthy and technical, see Karatzas and Shreve [13, Section 2.2]. That this procedure works is guaranteed by deep theorems initiated by Kolmogorov (the “consistency” theorem, and the “continuity” theorem). These say, respectively, that given a set of finite-dimensional distributions (FDDs), one can indeed construct a well-defined stochastic process with these FDDs, and under additional conditions on the moments of the increments (so, on $\mathbb{E}\left[\left|X_t-X_s\right|^\alpha\right], s \leq t$, for some positive $\alpha$ ), the process can be assumed to almost surely (so, with probability one) have continuous paths.
There are also a number of more direct constructions of BM. The first rigorous construction was by Norbert Wiener $[26,27]$ using Fourier series methods (his construction is outlined in Bass [1, Section 6.1]). Einstein, in 1905 [8], derived the transition density (the probability density $p(t, x)$ for the BM moving from 0 to $x$ in time $t$ ) for BM by considering the molecular-kinetic theory of heat (as being composed of many random collisions of molecules). An approach similar in spirit to Wiener’s (so also based on Hilbert space theory, namely sets of orthonormal functions), which uses the so-called Haar functions, was carried out by Lévy [18] and later simplified by Ciesielski [5]. Such a construction can be found in Karatzas and Shreve [13, Section 2.3].
Yet another another proof for the existence of BM is based on the idea of a weak limit (so a convergence in distribution) of a sequence of random walks. This construction can be found in Karatzas and Shreve [13, Section 2.4]. It involves constructing a sequence of probability spaces such that the corresponding sequence of probability measures satisfies a property known as tightness. One has a sequence of processes $\left(X^n\right){n \in \mathbb{N}}$ which induce a sequence of tight probability measures on $(C([0, \infty)), \mathcal{B}(C([0, \infty))))$ (the space of continuous functions on $[0, \infty)$ equipped with the Borel $\sigma$-field on this space), such that there is a limiting measure $\mathbb{P}*$ (called Wiener measure) under which the coordinate mapping process $W_t(\omega):=\omega(t), t \geq 0$ on $C([0, \infty))$ is a standard, one-dimensional Brownian motion. This is a lengthy and technical construction, to say the least. It is also possible to construct a probability space on which all the random walks are defined and converge to BM almost surely, rather than merely in distribution, as shown by Knight .

随机微积分代写
数学代写|随机微积分代写STOCHASTIC CALCULUS代考|Brownian motion
在本课程中我们将重点关注的随机过程是布朗运动 (BM) $W=\left(W_t\right) t \geq 0$ (这是所有涉及具有连续样本路径的过程的随机演算 的其石)。简而言之,BM 是一个连续过程,具有平稳、独立、正态分布(或高斯)增量,均值为零,方差等于增量期间经过的时 间。也就是说,对于 $0 \leq s \leq t<\infty$ ,增量 $W_t-W_s$ 根据分布 $W_t-W_s \sim \mathrm{N}(0, t-s)$ 并且独立于 $W_s$ (而且,确实,独立于 整个历史 $\left.\left(W_u\right) 0 \leq u \leq s\right)$. 在这里和其他地方,我们将使用符号 $X \sim \mathrm{N}\left(m, s^2\right)$ 表示随机変量 $X$ 服从均值正态分布 $m$ 和方差 $s^2$
我们将很快给出 BM 的一些严格定义。BM 具有人们所夆望的所有典型属性:它是一个鞅和一个马尔可夫过程(以及具有连紓路 径),也可以被认为是缩放和加速随机游走的极限。然而,正如我们将看到的,BM 的路径是高度不规则的;它们是不可微分的, 并且在任何有限的时间间隔内都是无限长的。
数学代写|随机微积分代写STOCHASTIC CALCULUS代考|Remarks on existence of BM
关于存在的备注 BM. 的存在BM作为一个明确定义的数学对象是一个不平凡的问题,但我们不会关心这个问题一一我们将假设 $\mathrm{BM}$ 的存在,尽管我们现在就在哪里可以找到 BM 的结构做一些简短的评论。
一种显示存在的方法BM是写下过程的有限维分布(基于其增量的平稳性、独立性和正态性)必须是什么,然后在适当的可测空间 上构建概率恻度和过程,这样我们就可以获得规定的有限维分布。
这种技术是构建马尔可夫过程(其中 BM 是一个示例)的标准方法,并且可能冗长且技术性强,请参阅 Karatzas 和 Shreve [13,第 $2.2$ 节]。Kolmogorov 提出的深层定理(“一致性”定理和”连埠性”定理)保证了此过程的有效性。它们分别表示,给定一 组有限维分布 (FDD),确实可以用这些 FDD 构造一个明确定义的随机过程,并且在增量矩的附加条件下(因此,在 $\mathbb{E}\left[\left|X_t-X_s\right|^\alpha\right], s \leq t$ ,对于一些积极的 $\alpha$ ),可以假设该过程几乎肯定(因此,概率为 1 ) 具有连续路径。
BM 也有许多更直接的结构。第一个严格的构造是由 Norbert Wiener $[26,27]$ 使用傅立叶级数方法(他的构造在 Bass [1,第 $6.1$ 节] 中进行了概述) 。咾因斯坦在1905年 [8]推导了跃迁密度 (概率密度 $p(t, x)$ 对于 BM 从 0 移动到 $x$ 及时 $t$ ) 对于 BM,通过考虑 热的分子动力学理论 (由许多分子的随机碰噇组成)。Lévy [18] 实施了一种与 Wiener 的方法 (因此也甚于 Hilbert 空间理论, 即正交函数集) 在本质上类似的方法,该方法使用所调的 Haar 函数,由 Lévy [18] 进行,后来由 Ciesielski [5] 进行了简化。这 种结构可以在 Karatzas 和 Shreve [13,第 $2.3$ 节] 中找到。
BM 存在的另一个证明是基于随机游走序列的弱极限(因此分布收敛)的想法。这种结构可以在 Karatzas 和 Shreve [13,第 $2.4$ 节] 中找到。它涉及构建概率空间序列,使得相应的概率测度序列满足称为苭度的属性。一个有一系列的过程 $\left(X^n\right) n \in \mathbb{N}$ 这引起 了一手列严格的概率测量 $(C([0, \infty)), \mathcal{B}(C([0, \infty)))$ ) (连紏函数的空间 $[0, \infty)$ 配备了 Borel $\sigma$-这个空间上的场),这样就有一 个限制措施 $\mathbb{P} *$ (称为维妠测度) 下的坐标映射过程 $W_t(\omega):=\omega(t), t \geq 0$ 上 $C([0, \infty))$ 是标准的一维布朗运动。至少可以说, 这是一个几长的技术结构。还可以构建一个概率空间,在该空间上定义了所有随机游走并几夹肯定地收敛到 BM,而不是仅仅在分 布中,如 Knight 所示。
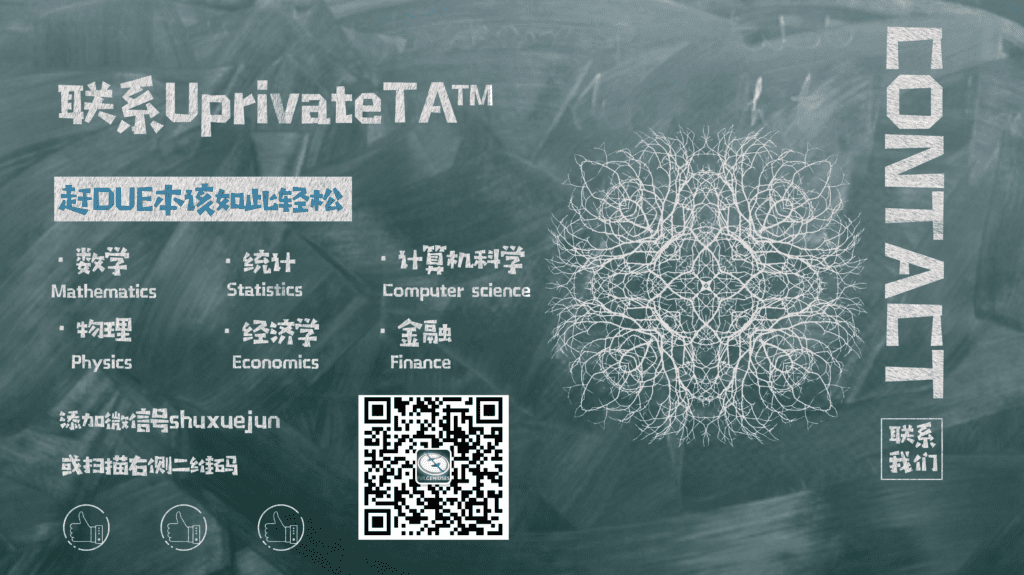
数学代写|随机微积分代写Stochastic Calculus代考 请认准UprivateTA™. UprivateTA™为您的留学生涯保驾护航。
微观经济学代写
微观经济学是主流经济学的一个分支,研究个人和企业在做出有关稀缺资源分配的决策时的行为以及这些个人和企业之间的相互作用。my-assignmentexpert™ 为您的留学生涯保驾护航 在数学Mathematics作业代写方面已经树立了自己的口碑, 保证靠谱, 高质且原创的数学Mathematics代写服务。我们的专家在图论代写Graph Theory代写方面经验极为丰富,各种图论代写Graph Theory相关的作业也就用不着 说。
线性代数代写
线性代数是数学的一个分支,涉及线性方程,如:线性图,如:以及它们在向量空间和通过矩阵的表示。线性代数是几乎所有数学领域的核心。
博弈论代写
现代博弈论始于约翰-冯-诺伊曼(John von Neumann)提出的两人零和博弈中的混合策略均衡的观点及其证明。冯-诺依曼的原始证明使用了关于连续映射到紧凑凸集的布劳威尔定点定理,这成为博弈论和数学经济学的标准方法。在他的论文之后,1944年,他与奥斯卡-莫根斯特恩(Oskar Morgenstern)共同撰写了《游戏和经济行为理论》一书,该书考虑了几个参与者的合作游戏。这本书的第二版提供了预期效用的公理理论,使数理统计学家和经济学家能够处理不确定性下的决策。
微积分代写
微积分,最初被称为无穷小微积分或 “无穷小的微积分”,是对连续变化的数学研究,就像几何学是对形状的研究,而代数是对算术运算的概括研究一样。
它有两个主要分支,微分和积分;微分涉及瞬时变化率和曲线的斜率,而积分涉及数量的累积,以及曲线下或曲线之间的面积。这两个分支通过微积分的基本定理相互联系,它们利用了无限序列和无限级数收敛到一个明确定义的极限的基本概念 。
计量经济学代写
什么是计量经济学?
计量经济学是统计学和数学模型的定量应用,使用数据来发展理论或测试经济学中的现有假设,并根据历史数据预测未来趋势。它对现实世界的数据进行统计试验,然后将结果与被测试的理论进行比较和对比。
根据你是对测试现有理论感兴趣,还是对利用现有数据在这些观察的基础上提出新的假设感兴趣,计量经济学可以细分为两大类:理论和应用。那些经常从事这种实践的人通常被称为计量经济学家。
MATLAB代写
MATLAB 是一种用于技术计算的高性能语言。它将计算、可视化和编程集成在一个易于使用的环境中,其中问题和解决方案以熟悉的数学符号表示。典型用途包括:数学和计算算法开发建模、仿真和原型制作数据分析、探索和可视化科学和工程图形应用程序开发,包括图形用户界面构建MATLAB 是一个交互式系统,其基本数据元素是一个不需要维度的数组。这使您可以解决许多技术计算问题,尤其是那些具有矩阵和向量公式的问题,而只需用 C 或 Fortran 等标量非交互式语言编写程序所需的时间的一小部分。MATLAB 名称代表矩阵实验室。MATLAB 最初的编写目的是提供对由 LINPACK 和 EISPACK 项目开发的矩阵软件的轻松访问,这两个项目共同代表了矩阵计算软件的最新技术。MATLAB 经过多年的发展,得到了许多用户的投入。在大学环境中,它是数学、工程和科学入门和高级课程的标准教学工具。在工业领域,MATLAB 是高效研究、开发和分析的首选工具。MATLAB 具有一系列称为工具箱的特定于应用程序的解决方案。对于大多数 MATLAB 用户来说非常重要,工具箱允许您学习和应用专业技术。工具箱是 MATLAB 函数(M 文件)的综合集合,可扩展 MATLAB 环境以解决特定类别的问题。可用工具箱的领域包括信号处理、控制系统、神经网络、模糊逻辑、小波、仿真等。