
如果你也在 怎样代写机器学习Machine Learning KIT315这个学科遇到相关的难题,请随时右上角联系我们的24/7代写客服。机器学习Machine Learning是一个致力于理解和建立 “学习 “方法的研究领域,也就是说,利用数据来提高某些任务的性能的方法。机器学习算法基于样本数据(称为训练数据)建立模型,以便在没有明确编程的情况下做出预测或决定。机器学习算法被广泛用于各种应用,如医学、电子邮件过滤、语音识别和计算机视觉,在这些应用中,开发传统算法来执行所需任务是困难的或不可行的。
机器学习Machine Learning程序可以在没有明确编程的情况下执行任务。它涉及到计算机从提供的数据中学习,从而执行某些任务。对于分配给计算机的简单任务,有可能通过编程算法告诉机器如何执行解决手头问题所需的所有步骤;就计算机而言,不需要学习。对于更高级的任务,由人类手动创建所需的算法可能是一个挑战。在实践中,帮助机器开发自己的算法,而不是让人类程序员指定每一个需要的步骤,可能会变得更加有效 。
机器学习Machine Learning代写,免费提交作业要求, 满意后付款,成绩80\%以下全额退款,安全省心无顾虑。专业硕 博写手团队,所有订单可靠准时,保证 100% 原创。 最高质量的机器学习Machine Learning作业代写,服务覆盖北美、欧洲、澳洲等 国家。 在代写价格方面,考虑到同学们的经济条件,在保障代写质量的前提下,我们为客户提供最合理的价格。 由于作业种类很多,同时其中的大部分作业在字数上都没有具体要求,因此机器学习Machine Learning作业代写的价格不固定。通常在专家查看完作业要求之后会给出报价。作业难度和截止日期对价格也有很大的影响。
avatest™帮您通过考试
avatest™的各个学科专家已帮了学生顺利通过达上千场考试。我们保证您快速准时完成各时长和类型的考试,包括in class、take home、online、proctor。写手整理各样的资源来或按照您学校的资料教您,创造模拟试题,提供所有的问题例子,以保证您在真实考试中取得的通过率是85%以上。如果您有即将到来的每周、季考、期中或期末考试,我们都能帮助您!
在不断发展的过程中,avatest™如今已经成长为论文代写,留学生作业代写服务行业的翘楚和国际领先的教育集团。全体成员以诚信为圆心,以专业为半径,以贴心的服务时刻陪伴着您, 用专业的力量帮助国外学子取得学业上的成功。
•最快12小时交付
•200+ 英语母语导师
•70分以下全额退款
想知道您作业确定的价格吗? 免费下单以相关学科的专家能了解具体的要求之后在1-3个小时就提出价格。专家的 报价比上列的价格能便宜好几倍。
我们在计算机Quantum computer代写方面已经树立了自己的口碑, 保证靠谱, 高质且原创的计算机Quantum computer代写服务。我们的专家在机器学习Machine Learning代写方面经验极为丰富,各种机器学习Machine Learning相关的作业也就用不着 说。

计算机代写|机器学习代写Machine Learning代考|Application: Bluetooth BLE Greenhouse Monitor
In this section, we provide the basic framework for the robot control algorithm. The control algorithm will read the IR sensors attached to the Arduino Nano 33 ANALOG IN (pins 0–2).
In response to the wall placement detected, the algorithm will render signals to turn the robot to avoid the maze walls. Provided in Fig. 3.16 is a truth table that shows all possibilities of maze placement that the robot might encounter. A detected wall is represented with a logic one. An asserted motor action is also represented with a logic one. As previously mentioned,due to the physical placement of the sensor array on the trailing edge of the robot, the sensor detecting maze walls to the right of the robot is physically located on the left side of the robot and vice versa.
Given the interface circuit used, the robot motors may only be moved in the forward direction. To render a left turn, the left motor is stopped and the right motor is asserted until the robot completes the turn. To render a right turn, the opposite action is required.
The task in writing the control algorithm is to take the UML activity diagram provided in Fig. 3.15 and the actions specified in the robot action truth table Fig. 3.16 and transform both into an Arduino sketch. This may seem formidable but we take it a step at a time.
The control algorithm begins with Arduino Nano 33 pin definitions. Variables are then declared for the readings from the three IR sensors. The two required Arduino functions follow: setup() and loop(). In the setup() function, Arduino Nano 33 pins are declared as output. The loop() begins by reading the current value of the three IR sensors. The 512 value corresponds to a particular IR sensor range. This value may be adjusted to change the range at which the maze wall is detected. The read of the IR sensors is followed by an eight part
if–else if statement. The statement contains a part for each row of the truth table provided in Fig. 3.16. For a given configuration of sensed walls, the appropriate wall detection LEDs are illuminated followed by commands to activate the motors (analogWrite) and illuminate the appropriate turn signals. The analogWrite command issues a signal from 0 to 3.3 VDC by sending a constant from 0 to 255 using pulse width modulation (PWM) techniques. The turn signal commands provide to actions: the appropriate turns signals are flashed and a 1.5 s total delay is provided. This provides the robot 1.5 s to render a turn. This delay may need to be adjusted during the testing phase.
计算机代写|机器学习代写Machine Learning代考|Overview
With the introduction to the Arduino IDE (Chap. 1), the Nano 33 BLE Sense (Chap. 2), and interface techniques (Chap. 3) complete; we concentrate on Artificial Intelligence (AI) and Machine Learning (ML) concepts and applications for microcontroller–based systems for the remainder of the book. In a recent release, the Arduino Team stated “Arduino is on a mission to make machine learning simple enough for everyone to use [https://www.blog.arduino.cc].” Those acquainted with AI and ML concepts might counter these concepts are most appropriate for more powerful computing platforms. However, recent developments have allowed certain AI and ML applications to be executed on microcontrollers once they have been trained.
We will see the training task, in certain applications, may also be accomplished on a microcontroller. Furthermore, we explore applications that lend themselves to remote, battery operated microcontroller–based AI and ML applications [4]. In the remainder of the book we limit our discussions to AI and ML techniques specifically for microcontrollers. The intent is to introduce the concepts and allow you to practice on low cost, accessible Arduino hardware and software.Figure 4.1 illustrates the relationship between Artificial Intelligence, Machine Learning, and Deep Learning. The goal of Artificial Intelligence is for computing machinery to imitate and mimic intelligent human behavior. Some trace the origins of AI back to 1300 BCE [4].We limit our historical review to AI developments within the 20th century and forward. Following a brief historical review, this chapter explores the concept of K Nearest Neighbors (KNN) and Decision Tree classification techniques.
Within the realm of AI, we explore Fuzzy Logic in Chap. 5. The overall goal of fuzzy logic is to control a system using a series of rule statements of the form “IF–THEN.” The input conditions of the “IF” statement, the antecedents, are obtained by taking precise, crisp input information from input sensors and transducers and mapping them to fuzzy input linguistic (word) variables. This is called the fuzzification step. The “THEN” portion of the rule, the consequences, are the control commands back to the system. Again, linguistic variables are used to describe the control output. The control output is defuzzified to obtain precise, crisp output control signals. The mapping of inputs to outputs via the “IF–THEN” statements are provided by the system designer.

机器学习代写
计算机代写|机器学习代写Machine Learning代考|Application: Bluetooth BLE Greenhouse Monitor
在本节中,我们提供了机器人控制算法的基本框架。控制算法将读取连接到 Arduino Nano 33 ANALOG IN(引脚 0-2)的红外传感器。
作为对检测到的墙放置的响应,该算法将呈现信号以转动机器人以避开迷宫墙。图 3.16 提供了一个真值表,显示了机器人可能遇到的迷宫放置的所有可能性。检测到的墙用逻辑一表示。断言的电机动作也用逻辑 1 表示。如前所述,由于传感器阵列在机器人后缘的物理位置,机器人右侧的传感器检测迷宫墙实际上位于机器人的左侧,反之亦然。
鉴于所使用的接口电路,机器人电机只能向前移动。要左转,左电机停止,右电机启动,直到机器人完成转弯。要右转,需要相反的动作。
编写控制算法的任务是采用图 3.15 中提供的 UML 活动图和图 3.16 机器人动作真值表中指定的动作,并将两者转换为 Arduino 草图。这可能看起来很艰巨,但我们一次又一次地迈出了一步。
控制算法从 Arduino Nano 33 引脚定义开始。然后为三个红外传感器的读数声明变量。两个必需的 Arduino 函数如下:setup() 和 loop()。在 setup() 函数中,Arduino Nano 33 引脚被声明为输出。loop() 首先读取三个红外传感器的当前值。512 值对应于特定的 IR 传感器范围。可以调整此值以更改检测到迷宫墙的范围。红外传感器的读取之后是八个部分
if-else if 语句。该语句包含图 3.16 中提供的真值表的每一行的一部分。对于感应墙壁的给定配置,相应的墙壁检测 LED 会亮起,然后发出命令以激活电机 (analogWrite) 并点亮相应的转向信号灯。analogWrite 命令通过使用脉冲宽度调制 (PWM) 技术发送 0 到 255 之间的常数来发出 0 到 3.3 VDC 的信号。转向信号命令提供动作:闪烁适当的转向信号并提供 1.5 秒的总延迟。这为机器人提供了 1.5 秒的转弯时间。在测试阶段可能需要调整此延迟。
计算机代写|机器学习代写Machine Learning代考|Overview
随着 Arduino IDE(第 1 章)、Nano 33 BLE Sense(第 2 章)和接口技术(第 3 章)的介绍完成;在本书的其余部分,我们将重点介绍人工智能 (AI) 和机器学习 (ML) 的概念以及基于微控制器的系统的应用。在最近的一次发布中,Arduino 团队表示“Arduino 的使命是让机器学习变得足够简单,让每个人都能使用 [https://www.blog.arduino.cc]。” 那些熟悉 AI 和 ML 概念的人可能会反驳这些概念最适合更强大的计算平台。然而,最近的发展允许某些 AI 和 ML 应用程序在经过训练后可以在微控制器上执行。
我们将看到训练任务,在某些应用中,也可能在微控制器上完成。此外,我们探索了适合远程、电池供电的基于微控制器的 AI 和 ML 应用程序的应用程序 [4]。在本书的其余部分,我们将讨论限制在专用于微控制器的 AI 和 ML 技术上。目的是介绍概念并允许您在低成本、易于访问的 Arduino 硬件和软件上进行练习。图 4.1 说明了人工智能、机器学习和深度学习之间的关系。人工智能的目标是让计算机器模仿和模仿人类的智能行为。有些人将 AI 的起源追溯到公元前 1300 年 [4]。我们将历史回顾限制在 20 世纪及以后的 AI 发展。
在人工智能领域,我们在第 1 章探讨了模糊逻辑。5. 模糊逻辑的总体目标是使用一系列“IF-THEN”形式的规则语句来控制系统。“IF”语句的输入条件,即前提,是通过从输入传感器和转换器获取精确、清晰的输入信息并将它们映射到模糊输入语言(单词)变量而获得的。这称为模糊化步骤。规则的“THEN”部分,即结果,是返回系统的控制命令。同样,语言变量用于描述控制输出。控制输出经过去模糊处理以获得精确、清晰的输出控制信号。通过“IF–THEN”语句将输入映射到输出由系统设计人员提供。
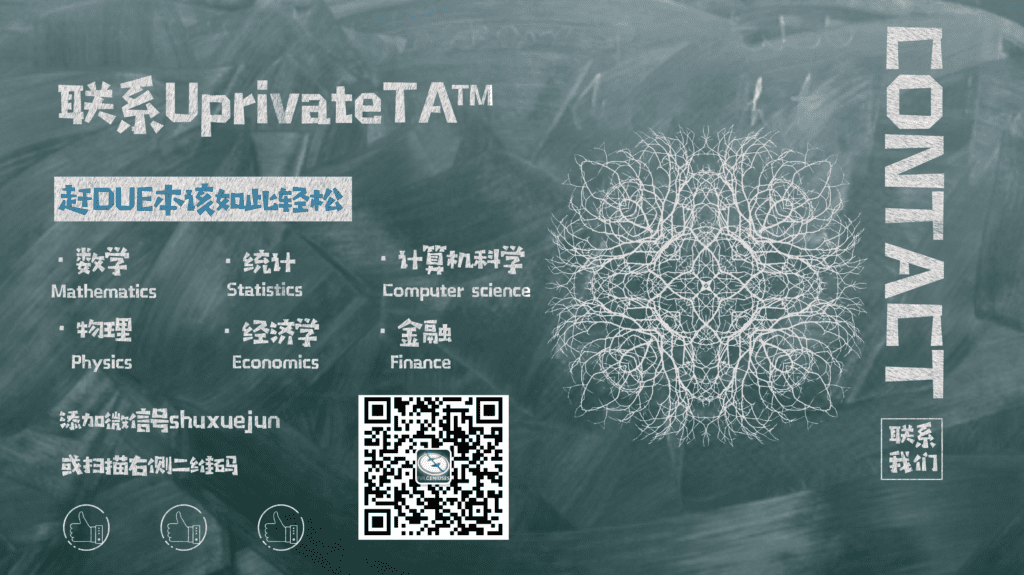
计算机代写|机器学习代写Machine Learning代考 请认准UprivateTA™. UprivateTA™为您的留学生涯保驾护航。
微观经济学代写
微观经济学是主流经济学的一个分支,研究个人和企业在做出有关稀缺资源分配的决策时的行为以及这些个人和企业之间的相互作用。my-assignmentexpert™ 为您的留学生涯保驾护航 在数学Mathematics作业代写方面已经树立了自己的口碑, 保证靠谱, 高质且原创的数学Mathematics代写服务。我们的专家在图论代写Graph Theory代写方面经验极为丰富,各种图论代写Graph Theory相关的作业也就用不着 说。
线性代数代写
线性代数是数学的一个分支,涉及线性方程,如:线性图,如:以及它们在向量空间和通过矩阵的表示。线性代数是几乎所有数学领域的核心。
博弈论代写
现代博弈论始于约翰-冯-诺伊曼(John von Neumann)提出的两人零和博弈中的混合策略均衡的观点及其证明。冯-诺依曼的原始证明使用了关于连续映射到紧凑凸集的布劳威尔定点定理,这成为博弈论和数学经济学的标准方法。在他的论文之后,1944年,他与奥斯卡-莫根斯特恩(Oskar Morgenstern)共同撰写了《游戏和经济行为理论》一书,该书考虑了几个参与者的合作游戏。这本书的第二版提供了预期效用的公理理论,使数理统计学家和经济学家能够处理不确定性下的决策。
微积分代写
微积分,最初被称为无穷小微积分或 “无穷小的微积分”,是对连续变化的数学研究,就像几何学是对形状的研究,而代数是对算术运算的概括研究一样。
它有两个主要分支,微分和积分;微分涉及瞬时变化率和曲线的斜率,而积分涉及数量的累积,以及曲线下或曲线之间的面积。这两个分支通过微积分的基本定理相互联系,它们利用了无限序列和无限级数收敛到一个明确定义的极限的基本概念 。
计量经济学代写
什么是计量经济学?
计量经济学是统计学和数学模型的定量应用,使用数据来发展理论或测试经济学中的现有假设,并根据历史数据预测未来趋势。它对现实世界的数据进行统计试验,然后将结果与被测试的理论进行比较和对比。
根据你是对测试现有理论感兴趣,还是对利用现有数据在这些观察的基础上提出新的假设感兴趣,计量经济学可以细分为两大类:理论和应用。那些经常从事这种实践的人通常被称为计量经济学家。
MATLAB代写
MATLAB 是一种用于技术计算的高性能语言。它将计算、可视化和编程集成在一个易于使用的环境中,其中问题和解决方案以熟悉的数学符号表示。典型用途包括:数学和计算算法开发建模、仿真和原型制作数据分析、探索和可视化科学和工程图形应用程序开发,包括图形用户界面构建MATLAB 是一个交互式系统,其基本数据元素是一个不需要维度的数组。这使您可以解决许多技术计算问题,尤其是那些具有矩阵和向量公式的问题,而只需用 C 或 Fortran 等标量非交互式语言编写程序所需的时间的一小部分。MATLAB 名称代表矩阵实验室。MATLAB 最初的编写目的是提供对由 LINPACK 和 EISPACK 项目开发的矩阵软件的轻松访问,这两个项目共同代表了矩阵计算软件的最新技术。MATLAB 经过多年的发展,得到了许多用户的投入。在大学环境中,它是数学、工程和科学入门和高级课程的标准教学工具。在工业领域,MATLAB 是高效研究、开发和分析的首选工具。MATLAB 具有一系列称为工具箱的特定于应用程序的解决方案。对于大多数 MATLAB 用户来说非常重要,工具箱允许您学习和应用专业技术。工具箱是 MATLAB 函数(M 文件)的综合集合,可扩展 MATLAB 环境以解决特定类别的问题。可用工具箱的领域包括信号处理、控制系统、神经网络、模糊逻辑、小波、仿真等。