
如果你也在 怎样代写线性回归Linear Regression 这个学科遇到相关的难题,请随时右上角联系我们的24/7代写客服。线性回归Linear Regression在统计学中,是对标量响应和一个或多个解释变量(也称为因变量和自变量)之间的关系进行建模的一种线性方法。一个解释变量的情况被称为简单线性回归;对于一个以上的解释变量,这一过程被称为多元线性回归。这一术语不同于多元线性回归,在多元线性回归中,预测的是多个相关的因变量,而不是一个标量变量。
线性回归Linear Regression在线性回归中,关系是用线性预测函数建模的,其未知的模型参数是根据数据估计的。最常见的是,假设给定解释变量(或预测因子)值的响应的条件平均值是这些值的仿生函数;不太常见的是,使用条件中位数或其他一些量化指标。像所有形式的回归分析一样,线性回归关注的是给定预测因子值的反应的条件概率分布,而不是所有这些变量的联合概率分布,这是多元分析的领域。
线性回归Linear Regression代写,免费提交作业要求, 满意后付款,成绩80\%以下全额退款,安全省心无顾虑。专业硕 博写手团队,所有订单可靠准时,保证 100% 原创。最高质量的线性回归Linear Regression作业代写,服务覆盖北美、欧洲、澳洲等 国家。 在代写价格方面,考虑到同学们的经济条件,在保障代写质量的前提下,我们为客户提供最合理的价格。 由于作业种类很多,同时其中的大部分作业在字数上都没有具体要求,因此线性回归Linear Regression作业代写的价格不固定。通常在专家查看完作业要求之后会给出报价。作业难度和截止日期对价格也有很大的影响。
avatest™帮您通过考试
avatest™的各个学科专家已帮了学生顺利通过达上千场考试。我们保证您快速准时完成各时长和类型的考试,包括in class、take home、online、proctor。写手整理各样的资源来或按照您学校的资料教您,创造模拟试题,提供所有的问题例子,以保证您在真实考试中取得的通过率是85%以上。如果您有即将到来的每周、季考、期中或期末考试,我们都能帮助您!
在不断发展的过程中,avatest™如今已经成长为论文代写,留学生作业代写服务行业的翘楚和国际领先的教育集团。全体成员以诚信为圆心,以专业为半径,以贴心的服务时刻陪伴着您, 用专业的力量帮助国外学子取得学业上的成功。
•最快12小时交付
•200+ 英语母语导师
•70分以下全额退款
想知道您作业确定的价格吗? 免费下单以相关学科的专家能了解具体的要求之后在1-3个小时就提出价格。专家的 报价比上列的价格能便宜好几倍。
我们在统计Statistics代写方面已经树立了自己的口碑, 保证靠谱, 高质且原创的统计Statistics代写服务。我们的专家在线性回归Linear Regression代写方面经验极为丰富,各种线性回归Linear Regression相关的作业也就用不着说。

统计代写|线性回归代写Linear Regression代考|Hypotheses Concerning One of the Terms
Obtaining information on one of the terms may be of interest. Can we do as well, understanding the mean function for Fuel if we delete the Tax variable? This amounts to the following hypothesis test of
$$
\begin{aligned}
& \mathrm{NH}: \quad \beta_1=0, \quad \beta_0, \beta_2, \beta_3, \beta_4 \text { arbitrary } \
& \mathrm{AH}: \quad \beta_1 \neq 0, \quad \beta_0, \beta_2, \beta_3, \beta_4 \text { arbitrary } \
&
\end{aligned}
$$
The following procedure can be used. First, fit the mean function that excludes the term for Tax and get the residual sum of squares for this smaller mean function.
Then fit again, this time including Tax, and once again get the residual sum of squares. Subtracting the residual sum of squares for the larger mean function from the residual sum of squares for the smaller mean function will give the sum of squares for regression on Tax after adjusting for the terms that are in both mean functions, Dlic, Income and $\log$ (Miles). Here is a summary of the computations that are needed:
\begin{tabular}{lcccccc}
& Df & SS & MS & F & Pr $(>F)$ \
Excluding Tax & 47 & 211964 & & & & \
Including Tax & 46 & 193700 & & & & \
\hline Difference & 1 & 18264 & 18264 & 4.34 & 0.043
\end{tabular}
The row marked “Excluding Tax” gives the df and $R S S$ for the mean function without $\operatorname{Tax}$, and the next line gives these values for the larger mean function including Tax. The difference between these two given on the next line is the sum of squares explained by Tax after adjusting for the other terms in the mean function. The $F$-test is given by $F=(18,264 / 1) / \hat{\sigma}^2=4.34$, which, when compared to the $F$ distribution with $(1,46)$ df gives a significance level of about 0.04 . We thus have modest evidence that the coefficient for Tax is different from zero. This is called a partial $F$-test. Partial $F$-tests can be generalized to testing several coefficients to be zero, but we delay that generalization to Section 5.4 .
统计代写|线性回归代写Linear Regression代考|Relationship to the t-Statistic
Another reasonable procedure for testing the importance of Tax is simply to compare the estimate of the coefficient divided by its standard error to the $t\left(n-p^{\prime}\right)$ distribution. One can show that the square of this $t$-statistic is the same number of the $F$-statistic just computed, so these two procedures are identical. Therefore, the $t$-statistic tests hypothesis (3.22) concerning the importance of terms adjusted for all the other terms, not ignoring them.
From Table 3.3, the $t$-statistic for Tax is $t=-2.083$, and $t^2=(-2.083)^2=$ 4.34 , the same as the $F$-statistic we just computed. The significance level for $\operatorname{Tax}$ given in Table 3.3 also agrees with the significance level we just obtained for the $F$-test, and so the significance level reported is for the two-sided test. To test the hypothesis that $\beta_1=0$ against the one-sided alternative that $\beta_1<0$, we could again use the same $t$-value, but the significance level would be one-half of the value for the two-sided test.
A $t$-test that $\beta_j$ has a specific value versus a two-sided or one-sided alternative (with all other coefficients arbitrary) can be carried out as described in Section 2.8.
In Section 3.1 , we discussed adding a term to a simple regression mean function. The same general procedure can be used to add a term to any linear regression mean function. For the added-variable plot for a term, say $X_1$, plot the residuals from the regression of $Y$ on all the other $X$ ‘s versus the residuals for the regression of $X_1$ on all the other $X \mathrm{~s}$. One can show (Problem 3.2) that (1) the slope of the regression in the added-variable plot is the estimated coefficient for $X_1$ in the regression with all the terms, and (2) the $t$-test for testing the slope to the zero in the added-variable plot is essentially the same as the $t$-test for testing $\beta_1=0$ in the fit of the larger mean function, the only difference being a correction for degrees of freedom.

线性回归代写
统计代写|线性回归代写Linear Regression代考|Hypotheses Concerning One of the Terms
获取其中一个条款的信息可能会让你感兴趣。如果我们删除Tax变量,我们也能理解Fuel的均值函数吗?这相当于下面的假设检验
$$
\begin{aligned}
& \mathrm{NH}: \quad \beta_1=0, \quad \beta_0, \beta_2, \beta_3, \beta_4 \text { arbitrary } \
& \mathrm{AH}: \quad \beta_1 \neq 0, \quad \beta_0, \beta_2, \beta_3, \beta_4 \text { arbitrary } \
&
\end{aligned}
$$
可以使用以下程序。首先,拟合排除Tax项的均值函数,并得到这个较小均值函数的残差平方和。
然后再次拟合,这次包括了Tax,再一次得到残差平方和。从较小的平均函数的残差平方和中减去较大的平均函数的残差平方和,在调整了两个平均函数(Dlic, Income和$\log$ (Miles))中的项后,将得到关于Tax的回归的平方和。以下是所需计算的摘要:
\begin{tabular}{lcccccc}
& Df & SS & MS & F & Pr $(>F)$ \Excluding Tax & 47 & 211964 & & & &\Including Tax & 46 & 193700 & & & &\hline Difference & 1 & 18264 & 18264 & 4.34 & 0.043
\end{tabular}
标记为“不含税”的行给出了不含$\operatorname{Tax}$的均值函数的df和$R S S$,下一行给出了包含Tax的较大均值函数的这些值。下一行给出的这两者之间的差值是Tax解释的平方和在调整了均值函数中的其他项之后。$F$ -检验由$F=(18,264 / 1) / \hat{\sigma}^2=4.34$给出,当将$F$分布与$(1,46)$ df比较时,其显著性水平约为0.04。因此,我们有适度的证据表明,税收系数不同于零。这被称为部分$F$ -测试。偏$F$ -检验可以推广到检验几个系数为零,但我们将这种推广推迟到第5.4节。
统计代写|线性回归代写Linear Regression代考|Relationship to the t-Statistic
另一个检验税收重要性的合理方法是简单地将系数估计值除以其标准误差与$t\left(n-p^{\prime}\right)$分布进行比较。我们可以证明,这个$t$ -统计量的平方与刚刚计算的$F$ -统计量的平方是相同的,所以这两个过程是相同的。因此,$t$ -statistic检验假设(3.22),关于为所有其他项调整的项的重要性,而不是忽略它们。
从表3.3中,Tax的$t$ -统计量为$t=-2.083$和$t^2=(-2.083)^2=$ 4.34,与我们刚刚计算的$F$ -统计量相同。表3.3中给出的$\operatorname{Tax}$的显著性水平也与我们刚刚得到的$F$ -检验的显著性水平一致,因此报告的显著性水平为双侧检验。为了检验假设$\beta_1=0$与单侧替代方案$\beta_1<0$,我们可以再次使用相同的$t$ -值,但显著性水平将是双侧检验值的一半。
一个$t$ -测试,$\beta_j$有一个特定的值,而不是一个双侧或单侧的替代(与所有其他系数任意),可以进行如第2.8节所述。
在3.1节中,我们讨论了在简单回归均值函数中添加一项。同样的一般程序可以用于向任何线性回归平均函数中添加一项。对于一个项(例如$X_1$)的加变量图,绘制$Y$在所有其他$X$上的回归的残差与$X_1$在所有其他$X \mathrm{~s}$上的回归的残差。可以证明(问题3.2):(1)加变量图中回归的斜率是所有项回归中$X_1$的估计系数,(2)用于检验加变量图中到零的斜率的$t$ -检验本质上与用于检验较大均值函数拟合中的$\beta_1=0$的$t$ -检验相同,唯一的区别是自由度的修正。
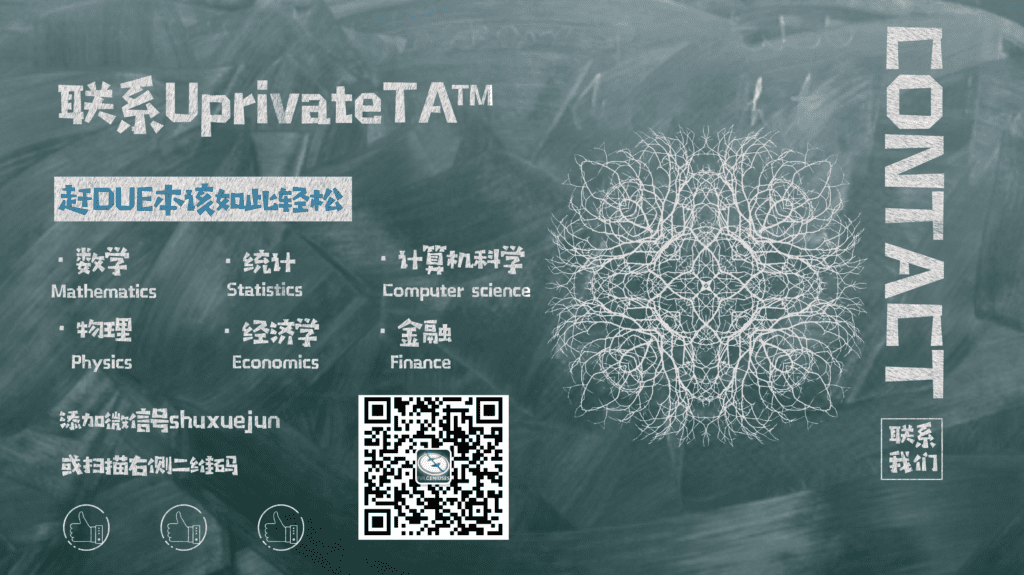
统计代写|线性回归代写Linear Regression代考 请认准UprivateTA™. UprivateTA™为您的留学生涯保驾护航。
微观经济学代写
微观经济学是主流经济学的一个分支,研究个人和企业在做出有关稀缺资源分配的决策时的行为以及这些个人和企业之间的相互作用。my-assignmentexpert™ 为您的留学生涯保驾护航 在数学Mathematics作业代写方面已经树立了自己的口碑, 保证靠谱, 高质且原创的数学Mathematics代写服务。我们的专家在图论代写Graph Theory代写方面经验极为丰富,各种图论代写Graph Theory相关的作业也就用不着 说。
线性代数代写
线性代数是数学的一个分支,涉及线性方程,如:线性图,如:以及它们在向量空间和通过矩阵的表示。线性代数是几乎所有数学领域的核心。
博弈论代写
现代博弈论始于约翰-冯-诺伊曼(John von Neumann)提出的两人零和博弈中的混合策略均衡的观点及其证明。冯-诺依曼的原始证明使用了关于连续映射到紧凑凸集的布劳威尔定点定理,这成为博弈论和数学经济学的标准方法。在他的论文之后,1944年,他与奥斯卡-莫根斯特恩(Oskar Morgenstern)共同撰写了《游戏和经济行为理论》一书,该书考虑了几个参与者的合作游戏。这本书的第二版提供了预期效用的公理理论,使数理统计学家和经济学家能够处理不确定性下的决策。
微积分代写
微积分,最初被称为无穷小微积分或 “无穷小的微积分”,是对连续变化的数学研究,就像几何学是对形状的研究,而代数是对算术运算的概括研究一样。
它有两个主要分支,微分和积分;微分涉及瞬时变化率和曲线的斜率,而积分涉及数量的累积,以及曲线下或曲线之间的面积。这两个分支通过微积分的基本定理相互联系,它们利用了无限序列和无限级数收敛到一个明确定义的极限的基本概念 。
计量经济学代写
什么是计量经济学?
计量经济学是统计学和数学模型的定量应用,使用数据来发展理论或测试经济学中的现有假设,并根据历史数据预测未来趋势。它对现实世界的数据进行统计试验,然后将结果与被测试的理论进行比较和对比。
根据你是对测试现有理论感兴趣,还是对利用现有数据在这些观察的基础上提出新的假设感兴趣,计量经济学可以细分为两大类:理论和应用。那些经常从事这种实践的人通常被称为计量经济学家。
MATLAB代写
MATLAB 是一种用于技术计算的高性能语言。它将计算、可视化和编程集成在一个易于使用的环境中,其中问题和解决方案以熟悉的数学符号表示。典型用途包括:数学和计算算法开发建模、仿真和原型制作数据分析、探索和可视化科学和工程图形应用程序开发,包括图形用户界面构建MATLAB 是一个交互式系统,其基本数据元素是一个不需要维度的数组。这使您可以解决许多技术计算问题,尤其是那些具有矩阵和向量公式的问题,而只需用 C 或 Fortran 等标量非交互式语言编写程序所需的时间的一小部分。MATLAB 名称代表矩阵实验室。MATLAB 最初的编写目的是提供对由 LINPACK 和 EISPACK 项目开发的矩阵软件的轻松访问,这两个项目共同代表了矩阵计算软件的最新技术。MATLAB 经过多年的发展,得到了许多用户的投入。在大学环境中,它是数学、工程和科学入门和高级课程的标准教学工具。在工业领域,MATLAB 是高效研究、开发和分析的首选工具。MATLAB 具有一系列称为工具箱的特定于应用程序的解决方案。对于大多数 MATLAB 用户来说非常重要,工具箱允许您学习和应用专业技术。工具箱是 MATLAB 函数(M 文件)的综合集合,可扩展 MATLAB 环境以解决特定类别的问题。可用工具箱的领域包括信号处理、控制系统、神经网络、模糊逻辑、小波、仿真等。