
如果你也在 怎样代写线性代数Linear algebra 学科遇到相关的难题,请随时右上角联系我们的24/7代写客服。线性代数Linear algebra是数学的一个基本领域,可以说是有史以来最强大的数学工具。
线性代数Linear algebra是商业、经济学、工程学、物理学、计算机科学、生态学、社会学、人口学和遗传学等领域的核心研究课题。举个线性代数的例子,只要看看Google搜索引擎就知道了,它依靠线性代数对搜索结果进行相关度排序。
线性代数Linear algebra代写,免费提交作业要求, 满意后付款,成绩80\%以下全额退款,安全省心无顾虑。专业硕 博写手团队,所有订单可靠准时,保证 100% 原创。 最高质量的线性代数Linear algebra作业代写,服务覆盖北美、欧洲、澳洲等 国家。 在代写价格方面,考虑到同学们的经济条件,在保障代写质量的前提下,我们为客户提供最合理的价格。 由于作业种类很多,同时其中的大部分作业在字数上都没有具体要求,因此线性代数Linear algebra作业代写的价格不固定。通常在专家查看完作业要求之后会给出报价。作业难度和截止日期对价格也有很大的影响。
avatest™帮您通过考试
avatest™的各个学科专家已帮了学生顺利通过达上千场考试。我们保证您快速准时完成各时长和类型的考试,包括in class、take home、online、proctor。写手整理各样的资源来或按照您学校的资料教您,创造模拟试题,提供所有的问题例子,以保证您在真实考试中取得的通过率是85%以上。如果您有即将到来的每周、季考、期中或期末考试,我们都能帮助您!
在不断发展的过程中,avatest™如今已经成长为论文代写,留学生作业代写服务行业的翘楚和国际领先的教育集团。全体成员以诚信为圆心,以专业为半径,以贴心的服务时刻陪伴着您, 用专业的力量帮助国外学子取得学业上的成功。
•最快12小时交付
•200+ 英语母语导师
•70分以下全额退款
想知道您作业确定的价格吗? 免费下单以相关学科的专家能了解具体的要求之后在1-3个小时就提出价格。专家的 报价比上列的价格能便宜好几倍。
我们在数学Mathematics代写方面已经树立了自己的口碑, 保证靠谱, 高质且原创的数学Mathematics代写服务。我们的专家在线性代数Linear algebra代写方面经验极为丰富,各种线性代数Linear algebra相关的作业也就用不着 说。

数学代写|线性代数代写Linear algebra代考|Properties of the range of a linear transformation
Note that the range of a linear transform $T: V \rightarrow W$ is composed of vectors in the arrival vector space $W$. (The vectors we arrive at in $W$ after applying $T$ to vectors in $V$.) Next we show that the range of $T$ is a subspace of the arrival vector space $W$.
Proposition (5.10). Let $V$ and $W$ be vector spaces and $T: V \rightarrow W$ be a linear transformation. The range or image of the transformation $T$ is a subspace of the arrival vector space $W$.
What does this mean?
The shaded part in $W$ in Fig. 5.16 is a subspace of $W$.
How do we prove this proposition?
Again, by using Proposition (3.7) of chapter 3:
A non-empty subset $S$ with vectors $\mathbf{u}$ and $\mathbf{v}$ is a subspace $\Leftrightarrow k \mathbf{u}+c \mathbf{v}$ is also in $S$.
Proof.
For a linear transform we have $T(\mathbf{O})=\mathbf{O}$, therefore $\mathbf{O}$ is in the set of $\operatorname{range}(T)$, which means the range is non-empty.
How do we prove that range $(T)$ is a subspace of the arrival space $W$ ?
By showing any linear combination is also in the range. Let $\mathbf{u}$ and $\mathbf{w}$ be vectors in $\operatorname{range}(T)$ then we need to show that
$$
k \mathbf{u}+c \mathbf{w} \text { is also in range }(T) \quad[k \text { and } c \text { are scalars }]
$$
Required to prove that the set $\operatorname{range}(T)$ is closed, which means that $k \mathbf{u}+c \mathbf{w}$ cannot escape from range $(T)$.
Since $\mathbf{u}$ and $\mathbf{w}$ are in $\operatorname{range}(T)$, there must exist input vectors $\mathbf{x}$ in $V$ and $\mathbf{y}$ in $V$ such that
$$
T(x)=\mathbf{u} \text { and } T(\mathbf{y})=\mathbf{w}
$$
数学代写|线性代数代写Linear algebra代考|Rank of a linear transformation (mapping)
The rank of a linear transformation tells us how much information has been transformed over and is measured as the dimension of the range. The rank also tells us whether information has been lost by the linear transform.
Definition (5.11). Let $T: V \rightarrow W$ be a linear transform (map) and range $(T)$ be the range. Then the dimension of range $(T)$ is called the rank of $T$ denoted $\operatorname{rank}(T)$ (Fig. 5.21).
Next we state one of the most important results of linear algebra. The proof of this theorem is given towards the end of the section because it is long and requires you to recall some definitions given in previous chapters.
Dimension theorem (also called the rank-nullity theorem) (5.12). Let $T: V \rightarrow W$ be a linear transformation from an $n$-dimensional vector space $V$ to a vector space $W$. Then
$$
\operatorname{rank}(T)+\operatorname{nullity}(T)=n
$$
What does this formula mean? It means
$$
\operatorname{dim}(\operatorname{range}(T))+\operatorname{dim}(\operatorname{ker}(T))=n
$$
where dim represents dimension. This is same as the dimension theorem (3.34) of chapter 3 . This suggests that adding the dimension of the range and kernel gives the dimension of the start vector space $V$. As stated earlier, this result says that all the information is contained in these two sets – kernel and range.
Why is the rank of a linear transformation important?
The rank gives us how much information has been carried over by the transform. If the rank of the linear transform $T: V \rightarrow W$ is equal to the dimension of the start vector space $V$ then all the information has been moved over and we can go back; that is, the linear transform has an inverse. We have:
- If $\operatorname{rank}(T)=\operatorname{dim}(V)$ then all the information has been carried over by $T$.
- If $\operatorname{rank}(T)<\operatorname{dim}(V)$ then some information has been lost by $T$.
- If $\operatorname{rank}(T)=0<\operatorname{dim}(V)$ then virtually all the information has been lost by $T$.

线性代数代写
数学代写|线性代数代写Linear algebra代考|Properties of the range of a linear transformation
注意,线性变换$T: V \rightarrow W$的范围是由到达向量空间$W$中的向量组成的。(将$T$应用于$V$中的向量后,我们到达$W$中的向量。)接下来我们证明$T$的范围是到达向量空间$W$的一个子空间。
提案(5.10)。设$V$和$W$是向量空间$T: V \rightarrow W$是线性变换。变换$T$的值域或像是到达向量空间$W$的子空间。
这是什么意思?
图5.16中$W$中的阴影部分为$W$的一个子空间。
我们如何证明这个命题?
再用第三章的命题(3.7):
具有向量$\mathbf{u}$和$\mathbf{v}$的非空子集$S$是子空间$\Leftrightarrow k \mathbf{u}+c \mathbf{v}$也在$S$中。
证明。
对于一个线性变换,我们有$T(\mathbf{O})=\mathbf{O}$,因此$\mathbf{O}$在$\operatorname{range}(T)$的集合中,这意味着值域是非空的。
我们如何证明值域$(T)$是到达空间$W$的一个子空间?
通过表示任意线性组合也在这个范围内。设$\mathbf{u}$和$\mathbf{w}$是$\operatorname{range}(T)$中的向量然后我们需要证明这一点
$$
k \mathbf{u}+c \mathbf{w} \text { is also in range }(T) \quad[k \text { and } c \text { are scalars }]
$$
需要证明集合$\operatorname{range}(T)$是封闭的,这意味着$k \mathbf{u}+c \mathbf{w}$不能从范围$(T)$中逃逸。
自从 $\mathbf{u}$ 和 $\mathbf{w}$ 我们在 $\operatorname{range}(T)$,必须存在输入向量 $\mathbf{x}$ 在 $V$ 和 $\mathbf{y}$ 在 $V$ 这样
$$
T(x)=\mathbf{u} \text { and } T(\mathbf{y})=\mathbf{w}
$$
数学代写|线性代数代写Linear algebra代考|Rank of a linear transformation (mapping)
线性变换的秩告诉我们有多少信息被变换了,并被测量为值域的维数。秩还告诉我们线性变换是否丢失了信息。
定义(5.11)。设$T: V \rightarrow W$为线性变换(映射),range $(T)$为范围。则范围$(T)$的维数称为$T$的秩,记为$\operatorname{rank}(T)$(图5.21)。
接下来我们陈述线性代数最重要的结果之一。这个定理的证明在本节的末尾给出,因为它很长,并且需要你回忆一下前面章节中给出的一些定义。
维数定理(也称为秩-零定理)(5.12)。设$T: V \rightarrow W$是一个线性变换从一个$n$维向量空间$V$到一个向量空间$W$。然后
$$
\operatorname{rank}(T)+\operatorname{nullity}(T)=n
$$
这个公式是什么意思?意思是
$$
\operatorname{dim}(\operatorname{range}(T))+\operatorname{dim}(\operatorname{ker}(T))=n
$$
其中dim表示维度。这与第三章的量纲定理(3.34)相同。这表明,将范围和核的维数相加,就得到了起始向量空间$V$的维数。如前所述,这个结果表明所有的信息都包含在这两个集合中——核和值域。
为什么线性变换的秩很重要?
这个秩告诉我们变换传递了多少信息。如果线性变换的秩$T: V \rightarrow W$等于起始向量空间的维数$V$那么所有的信息都被移动了,我们可以回去;也就是说,线性变换有一个逆函数。我们有:
如果是$\operatorname{rank}(T)=\operatorname{dim}(V)$,那么所有的信息都被$T$转移了。
如果$\operatorname{rank}(T)<\operatorname{dim}(V)$,那么一些信息已经被$T$丢失了。
如果是$\operatorname{rank}(T)=0<\operatorname{dim}(V)$,那么到$T$时,几乎所有的信息都丢失了。
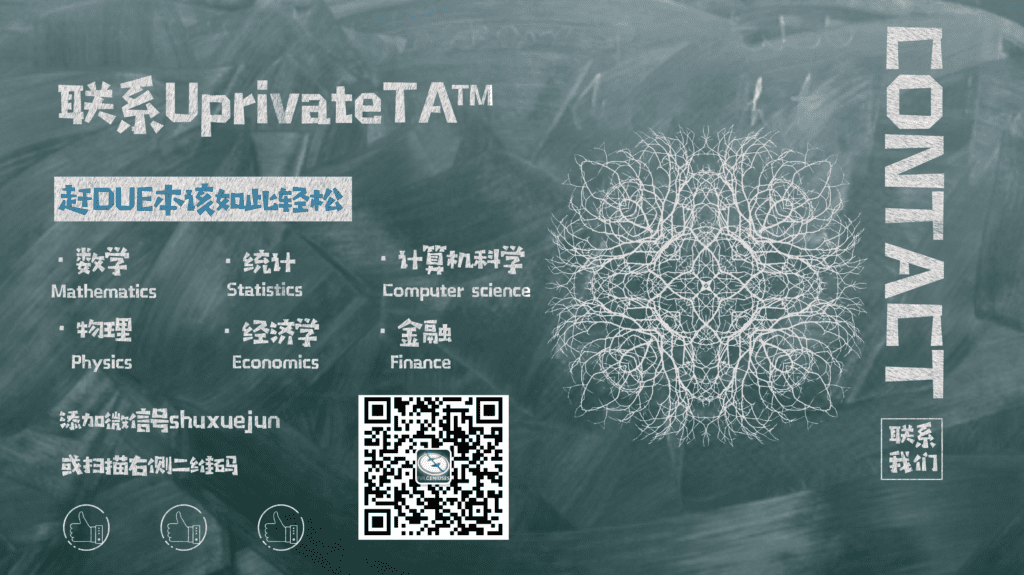
数学代写|线性代数代写Linear algebra代考 请认准UprivateTA™. UprivateTA™为您的留学生涯保驾护航。
微观经济学代写
微观经济学是主流经济学的一个分支,研究个人和企业在做出有关稀缺资源分配的决策时的行为以及这些个人和企业之间的相互作用。my-assignmentexpert™ 为您的留学生涯保驾护航 在数学Mathematics作业代写方面已经树立了自己的口碑, 保证靠谱, 高质且原创的数学Mathematics代写服务。我们的专家在图论代写Graph Theory代写方面经验极为丰富,各种图论代写Graph Theory相关的作业也就用不着 说。
线性代数代写
线性代数是数学的一个分支,涉及线性方程,如:线性图,如:以及它们在向量空间和通过矩阵的表示。线性代数是几乎所有数学领域的核心。
博弈论代写
现代博弈论始于约翰-冯-诺伊曼(John von Neumann)提出的两人零和博弈中的混合策略均衡的观点及其证明。冯-诺依曼的原始证明使用了关于连续映射到紧凑凸集的布劳威尔定点定理,这成为博弈论和数学经济学的标准方法。在他的论文之后,1944年,他与奥斯卡-莫根斯特恩(Oskar Morgenstern)共同撰写了《游戏和经济行为理论》一书,该书考虑了几个参与者的合作游戏。这本书的第二版提供了预期效用的公理理论,使数理统计学家和经济学家能够处理不确定性下的决策。
微积分代写
微积分,最初被称为无穷小微积分或 “无穷小的微积分”,是对连续变化的数学研究,就像几何学是对形状的研究,而代数是对算术运算的概括研究一样。
它有两个主要分支,微分和积分;微分涉及瞬时变化率和曲线的斜率,而积分涉及数量的累积,以及曲线下或曲线之间的面积。这两个分支通过微积分的基本定理相互联系,它们利用了无限序列和无限级数收敛到一个明确定义的极限的基本概念 。
计量经济学代写
什么是计量经济学?
计量经济学是统计学和数学模型的定量应用,使用数据来发展理论或测试经济学中的现有假设,并根据历史数据预测未来趋势。它对现实世界的数据进行统计试验,然后将结果与被测试的理论进行比较和对比。
根据你是对测试现有理论感兴趣,还是对利用现有数据在这些观察的基础上提出新的假设感兴趣,计量经济学可以细分为两大类:理论和应用。那些经常从事这种实践的人通常被称为计量经济学家。
MATLAB代写
MATLAB 是一种用于技术计算的高性能语言。它将计算、可视化和编程集成在一个易于使用的环境中,其中问题和解决方案以熟悉的数学符号表示。典型用途包括:数学和计算算法开发建模、仿真和原型制作数据分析、探索和可视化科学和工程图形应用程序开发,包括图形用户界面构建MATLAB 是一个交互式系统,其基本数据元素是一个不需要维度的数组。这使您可以解决许多技术计算问题,尤其是那些具有矩阵和向量公式的问题,而只需用 C 或 Fortran 等标量非交互式语言编写程序所需的时间的一小部分。MATLAB 名称代表矩阵实验室。MATLAB 最初的编写目的是提供对由 LINPACK 和 EISPACK 项目开发的矩阵软件的轻松访问,这两个项目共同代表了矩阵计算软件的最新技术。MATLAB 经过多年的发展,得到了许多用户的投入。在大学环境中,它是数学、工程和科学入门和高级课程的标准教学工具。在工业领域,MATLAB 是高效研究、开发和分析的首选工具。MATLAB 具有一系列称为工具箱的特定于应用程序的解决方案。对于大多数 MATLAB 用户来说非常重要,工具箱允许您学习和应用专业技术。工具箱是 MATLAB 函数(M 文件)的综合集合,可扩展 MATLAB 环境以解决特定类别的问题。可用工具箱的领域包括信号处理、控制系统、神经网络、模糊逻辑、小波、仿真等。