
如果你也在 怎样代写复杂网络Complex Network 这个学科遇到相关的难题,请随时右上角联系我们的24/7代写客服。复杂网络Complex Network在网络理论的背景下,复杂网络是指具有非微观拓扑特征的图(网络)–这些特征在简单的网络(如格子或随机图)中不会出现,但在代表真实系统的网络中经常出现。复杂网络的研究是一个年轻而活跃的科学研究领域(自2000年以来),主要受到现实世界网络的经验发现的启发,如计算机网络、生物网络、技术网络、大脑网络、气候网络和社会网络。
复杂网络Complex Network大多数社会、生物和技术网络显示出实质性的非微观拓扑特征,其元素之间的连接模式既不是纯粹的规则也不是纯粹的随机。这些特征包括学位分布的重尾、高聚类系数、顶点之间的同态性或异态性、社区结构和层次结构。在有向网络的情况下,这些特征还包括互惠性、三联体重要性概况和其他特征。相比之下,过去研究的许多网络的数学模型,如格子和随机图,并没有显示这些特征。最复杂的结构可以由具有中等数量相互作用的网络实现。这与中等概率获得最大信息含量(熵)的事实相对应。
复杂网络Complex Network代写,免费提交作业要求, 满意后付款,成绩80\%以下全额退款,安全省心无顾虑。专业硕 博写手团队,所有订单可靠准时,保证 100% 原创。最高质量的复杂网络Complex Network作业代写,服务覆盖北美、欧洲、澳洲等 国家。 在代写价格方面,考虑到同学们的经济条件,在保障代写质量的前提下,我们为客户提供最合理的价格。 由于作业种类很多,同时其中的大部分作业在字数上都没有具体要求,因此复杂网络Complex Network作业代写的价格不固定。通常在专家查看完作业要求之后会给出报价。作业难度和截止日期对价格也有很大的影响。
avatest™帮您通过考试
avatest™的各个学科专家已帮了学生顺利通过达上千场考试。我们保证您快速准时完成各时长和类型的考试,包括in class、take home、online、proctor。写手整理各样的资源来或按照您学校的资料教您,创造模拟试题,提供所有的问题例子,以保证您在真实考试中取得的通过率是85%以上。如果您有即将到来的每周、季考、期中或期末考试,我们都能帮助您!
在不断发展的过程中,avatest™如今已经成长为论文代写,留学生作业代写服务行业的翘楚和国际领先的教育集团。全体成员以诚信为圆心,以专业为半径,以贴心的服务时刻陪伴着您, 用专业的力量帮助国外学子取得学业上的成功。
•最快12小时交付
•200+ 英语母语导师
•70分以下全额退款
想知道您作业确定的价格吗? 免费下单以相关学科的专家能了解具体的要求之后在1-3个小时就提出价格。专家的 报价比上列的价格能便宜好几倍。
我们在统计Statistics代写方面已经树立了自己的口碑, 保证靠谱, 高质且原创的统计Statistics代写服务。我们的专家在复杂网络Complex Network代写方面经验极为丰富,各种复杂网络Complex Network相关的作业也就用不着说。

数据科学代写|复杂网络代写Complex Network代考|Graph Partitioning Using the Cavity Method
The statistical mechanics formulation of the q-partitioning problem is done via the following ferromagnetic Potts Hamiltonian:
$$
\mathcal{H}F({\sigma})=-\sum{i \neq j} J_{i j} \delta\left(\sigma_i, \sigma_j\right),
$$
where $J_{i j}$ is the ${0,1}$ adjacency matrix of the graph and $\sigma_i$ denotes the Potts spin variable with $\sigma_i \in{1,2, \ldots, q}$. Once one finds the ground state under the constraint $\sum_i \delta\left(\sigma_i, \tau\right)=N / q$ for all $\tau \in{1,2, \ldots, q}$, one can write the total number of cut edges $C$ in the system using the ground state energy $E_g$ of the above Hamiltonian (6.1):
$$
C_q=M+E_g=M\left(\frac{q-1}{q}-Q_q\right) .
$$
Note the difference to (5.5). Also note that the modularity of the q-partition $Q_q$ can be expressed via Hamiltonian (6.1) as
$$
Q_q=-\frac{\mathcal{H}_F}{M}-\frac{1}{q} .
$$
This expression is only valid for magnetization zero, i.e., an exact q-partition.
数据科学代写|复杂网络代写Complex Network代考|Cavity Method at Zero Temperature
The ground state energy of (6.1) can be calculated by applying the cavity method at zero temperature following the approach presented by Mezard and Parisi [8] in the formulation for a Potts model as presented by Braunstein et al. $[9,10]$ for coloring random graphs. The energy of a system of $N$ spins is written as dependent on a “cavity spin” $\sigma_1$ via the “cavity field” $\boldsymbol{h}1$ : $$ E^N\left(\sigma_1\right)=A-\sum{\tau=1}^q h_1^\tau \delta\left(\tau, \sigma_1\right)
$$
Note that $h_1^\tau$ takes only integer values, if $J_{i j}$ is composed of only ${0,1}$. The components of the cavity field $\boldsymbol{h}i$ denote the change in energy of the system with a change in spin $i$. In general, these are different from the “effective fields” $\sum_j J{i j} \sigma_j$ acting on spin $\sigma_i$, which are used to calculate the magnetization. Adding a new spin $\sigma_0$ connected to $\sigma_1$, the energy of the now $N+1$ spin system is a function of both $\sigma_1$ and $\sigma_0$ :
$$
E^{N+1}\left(\sigma_1, \sigma_0\right)=A-\sum_{\tau=1}^q h_1^\tau \delta\left(\tau, \sigma_1\right)-J_{10} \delta\left(\sigma_1, \sigma_0\right) .
$$
One can now write this expression in such a way that it only depends on the newly added cavity spin $\sigma_0$ :
$$
E^{N+1}\left(\sigma_0\right)=\min {\sigma_1} E^{N+1}\left(\sigma_1, \sigma_0\right) \equiv A-w\left(\boldsymbol{h}_1\right)-\sum{\tau=1}^q \hat{u}^\tau\left(J_{10}, \boldsymbol{h}_1\right) \delta\left(\tau, \sigma_0\right) .
$$
The functions $w$ and $\hat{u}$ take the following form:
$$
\begin{aligned}
w(\boldsymbol{h}) & =\max \left(h^1, \ldots, h^q\right), \
\hat{u}^\tau(J, \boldsymbol{h}) & =\max \left(h^1, \ldots, h^\tau+J, \ldots, h^q\right)-w(\boldsymbol{h}) .
\end{aligned}
$$
From (6.8) one sees that $\hat{u}^\tau(\boldsymbol{h})$ is one, whenever the $\tau$ th component of $\boldsymbol{h}$ is maximal with respect to all other components in $\boldsymbol{h}$ and zero otherwise. Due to possible degeneracy in the components of $\boldsymbol{h}$, the vector $\hat{u}(\boldsymbol{h})$ may have more than one non-zero entry and is never completely zero.

复杂网络代写
数据科学代写|复杂网络代写Complex Network代考|Graph Partitioning Using the Cavity Method
q划分问题的统计力学公式是通过以下铁磁波茨哈密顿量来完成的:
$$
\mathcal{H}F({\sigma})=-\sum{i \neq j} J_{i j} \delta\left(\sigma_i, \sigma_j\right),
$$
其中$J_{i j}$为图形的${0,1}$邻接矩阵,$\sigma_i$为Potts自旋变量$\sigma_i \in{1,2, \ldots, q}$。一旦找到所有$\tau \in{1,2, \ldots, q}$在约束$\sum_i \delta\left(\sigma_i, \tau\right)=N / q$下的基态,就可以利用上述哈密顿量(6.1)的基态能量$E_g$写出系统中切割边的总数$C$:
$$
C_q=M+E_g=M\left(\frac{q-1}{q}-Q_q\right) .
$$
请注意与(5.5)的区别。还要注意,q分区$Q_q$的模块化可以通过哈密顿量(6.1)表示为
$$
Q_q=-\frac{\mathcal{H}_F}{M}-\frac{1}{q} .
$$
这个表达式只适用于磁化强度为零的情况,即精确的q划分。
数据科学代写|复杂网络代写Complex Network代考|Cavity Method at Zero Temperature
(6.1)的基态能量可以按照Mezard和Parisi[8]在Braunstein等人$[9,10]$为随机图上色提出的Potts模型公式中提出的方法,在零温度下应用空腔法计算。一个$N$自旋系统的能量被写成依赖于“腔自旋”$\sigma_1$通过“腔场”$\boldsymbol{h}1$: $$ E^N\left(\sigma_1\right)=A-\sum{\tau=1}^q h_1^\tau \delta\left(\tau, \sigma_1\right)
$$
注意,如果$J_{i j}$只由${0,1}$组成,那么$h_1^\tau$只接受整数值。腔场的分量$\boldsymbol{h}i$表示系统的能量随自旋$i$的变化。一般来说,这些不同于作用于自旋$\sigma_i$的“有效场”$\sum_j J{i j} \sigma_j$,用于计算磁化强度。加入一个新的自旋$\sigma_0$连接到$\sigma_1$,现在的$N+1$自旋系统的能量是$\sigma_1$和$\sigma_0$的函数:
$$
E^{N+1}\left(\sigma_1, \sigma_0\right)=A-\sum_{\tau=1}^q h_1^\tau \delta\left(\tau, \sigma_1\right)-J_{10} \delta\left(\sigma_1, \sigma_0\right) .
$$
现在我们可以这样写这个表达式,它只依赖于新增加的腔自旋$\sigma_0$:
$$
E^{N+1}\left(\sigma_0\right)=\min {\sigma_1} E^{N+1}\left(\sigma_1, \sigma_0\right) \equiv A-w\left(\boldsymbol{h}1\right)-\sum{\tau=1}^q \hat{u}^\tau\left(J{10}, \boldsymbol{h}_1\right) \delta\left(\tau, \sigma_0\right) .
$$
$w$和$\hat{u}$函数的形式如下:
$$
\begin{aligned}
w(\boldsymbol{h}) & =\max \left(h^1, \ldots, h^q\right), \
\hat{u}^\tau(J, \boldsymbol{h}) & =\max \left(h^1, \ldots, h^\tau+J, \ldots, h^q\right)-w(\boldsymbol{h}) .
\end{aligned}
$$
从式(6.8)中可以看出,当$\boldsymbol{h}$的第$\tau$个分量相对于$\boldsymbol{h}$中的所有其他分量最大时,$\hat{u}^\tau(\boldsymbol{h})$为1,否则为零。由于$\boldsymbol{h}$的分量可能存在简并性,向量$\hat{u}(\boldsymbol{h})$可能有多个非零项,并且永远不会完全为零。
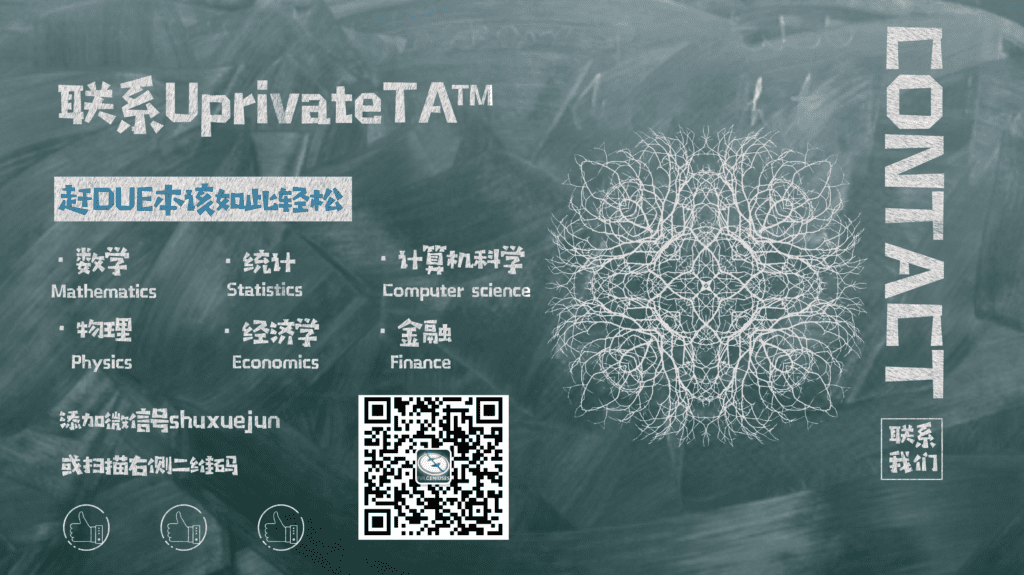
数据科学代写|复杂网络代写Complex Network代考 请认准UprivateTA™. UprivateTA™为您的留学生涯保驾护航。
微观经济学代写
微观经济学是主流经济学的一个分支,研究个人和企业在做出有关稀缺资源分配的决策时的行为以及这些个人和企业之间的相互作用。my-assignmentexpert™ 为您的留学生涯保驾护航 在数学Mathematics作业代写方面已经树立了自己的口碑, 保证靠谱, 高质且原创的数学Mathematics代写服务。我们的专家在图论代写Graph Theory代写方面经验极为丰富,各种图论代写Graph Theory相关的作业也就用不着 说。
线性代数代写
线性代数是数学的一个分支,涉及线性方程,如:线性图,如:以及它们在向量空间和通过矩阵的表示。线性代数是几乎所有数学领域的核心。
博弈论代写
现代博弈论始于约翰-冯-诺伊曼(John von Neumann)提出的两人零和博弈中的混合策略均衡的观点及其证明。冯-诺依曼的原始证明使用了关于连续映射到紧凑凸集的布劳威尔定点定理,这成为博弈论和数学经济学的标准方法。在他的论文之后,1944年,他与奥斯卡-莫根斯特恩(Oskar Morgenstern)共同撰写了《游戏和经济行为理论》一书,该书考虑了几个参与者的合作游戏。这本书的第二版提供了预期效用的公理理论,使数理统计学家和经济学家能够处理不确定性下的决策。
微积分代写
微积分,最初被称为无穷小微积分或 “无穷小的微积分”,是对连续变化的数学研究,就像几何学是对形状的研究,而代数是对算术运算的概括研究一样。
它有两个主要分支,微分和积分;微分涉及瞬时变化率和曲线的斜率,而积分涉及数量的累积,以及曲线下或曲线之间的面积。这两个分支通过微积分的基本定理相互联系,它们利用了无限序列和无限级数收敛到一个明确定义的极限的基本概念 。
计量经济学代写
什么是计量经济学?
计量经济学是统计学和数学模型的定量应用,使用数据来发展理论或测试经济学中的现有假设,并根据历史数据预测未来趋势。它对现实世界的数据进行统计试验,然后将结果与被测试的理论进行比较和对比。
根据你是对测试现有理论感兴趣,还是对利用现有数据在这些观察的基础上提出新的假设感兴趣,计量经济学可以细分为两大类:理论和应用。那些经常从事这种实践的人通常被称为计量经济学家。
MATLAB代写
MATLAB 是一种用于技术计算的高性能语言。它将计算、可视化和编程集成在一个易于使用的环境中,其中问题和解决方案以熟悉的数学符号表示。典型用途包括:数学和计算算法开发建模、仿真和原型制作数据分析、探索和可视化科学和工程图形应用程序开发,包括图形用户界面构建MATLAB 是一个交互式系统,其基本数据元素是一个不需要维度的数组。这使您可以解决许多技术计算问题,尤其是那些具有矩阵和向量公式的问题,而只需用 C 或 Fortran 等标量非交互式语言编写程序所需的时间的一小部分。MATLAB 名称代表矩阵实验室。MATLAB 最初的编写目的是提供对由 LINPACK 和 EISPACK 项目开发的矩阵软件的轻松访问,这两个项目共同代表了矩阵计算软件的最新技术。MATLAB 经过多年的发展,得到了许多用户的投入。在大学环境中,它是数学、工程和科学入门和高级课程的标准教学工具。在工业领域,MATLAB 是高效研究、开发和分析的首选工具。MATLAB 具有一系列称为工具箱的特定于应用程序的解决方案。对于大多数 MATLAB 用户来说非常重要,工具箱允许您学习和应用专业技术。工具箱是 MATLAB 函数(M 文件)的综合集合,可扩展 MATLAB 环境以解决特定类别的问题。可用工具箱的领域包括信号处理、控制系统、神经网络、模糊逻辑、小波、仿真等。